Pipeline abnormality type automatic detection method based on deep learning
An abnormal type, deep learning technology, applied in the fields of computer vision, computer image processing and deep learning, can solve problems such as pipeline abnormalities, inability to be put into large-scale production, and recognition accuracy dependence.
- Summary
- Abstract
- Description
- Claims
- Application Information
AI Technical Summary
Problems solved by technology
Method used
Image
Examples
Embodiment Construction
[0026] In order to make the object, technical solution and advantages of the present invention more clear, the present invention will be described in detail below with reference to the accompanying drawings and examples. Apparently, the described implementation is only a part of the embodiments of the present invention, rather than an exhaustive list of all the embodiments. And in the case of no conflict, the implementations in this description and the features in the embodiments can be combined with each other.
[0027] By using deep learning technology, especially the Convolutional Neural Network (CNN), which has shined in the field of computer vision in recent years, computers have achieved considerable accuracy in the field of image recognition and classification. Therefore, it is possible to use the existing, artificially marked pipeline anomaly images to train the CNN model to extract the features of the pipeline anomalies and identify the abnormal type of the drainage p...
PUM
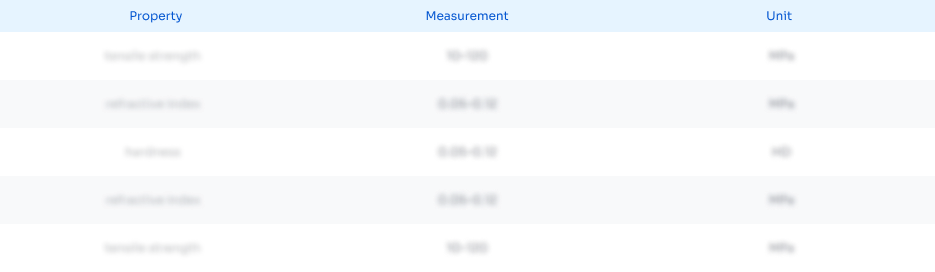
Abstract
Description
Claims
Application Information
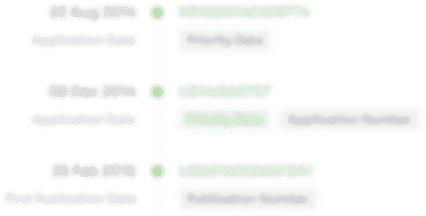
- R&D Engineer
- R&D Manager
- IP Professional
- Industry Leading Data Capabilities
- Powerful AI technology
- Patent DNA Extraction
Browse by: Latest US Patents, China's latest patents, Technical Efficacy Thesaurus, Application Domain, Technology Topic, Popular Technical Reports.
© 2024 PatSnap. All rights reserved.Legal|Privacy policy|Modern Slavery Act Transparency Statement|Sitemap|About US| Contact US: help@patsnap.com