A Knowledge Graph Representation Learning Method Based on Cosine Metric Rule
A technology of knowledge graph and learning method, applied in knowledge expression, character and pattern recognition, unstructured text data retrieval, etc. The effect of improving model performance
- Summary
- Abstract
- Description
- Claims
- Application Information
AI Technical Summary
Problems solved by technology
Method used
Image
Examples
Embodiment Construction
[0040] In order to make the object, technical solution and advantages of the present invention clearer, the present invention will be further described in detail below in combination with specific examples and with reference to the accompanying drawings.
[0041] The invention discloses a knowledge map representation learning method based on cosine metric rules, such as figure 1 As shown, firstly, the entities and relationships in the knowledge graph are randomly embedded into two vector spaces; secondly, using the statistical rules of candidate entities, the triple sets and candidate entity vector sets corresponding to the statistical correlations are counted, and randomly generated according to the candidate entity vector sets Wrong entity vector set; again use the cosine similarity to construct the scoring function of the target vector and the candidate entity, and evaluate the candidate entity; finally, use the loss function to uniformly train the candidate entity vector and ...
PUM
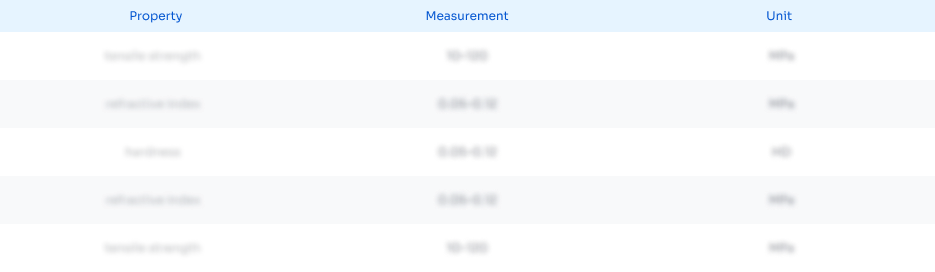
Abstract
Description
Claims
Application Information
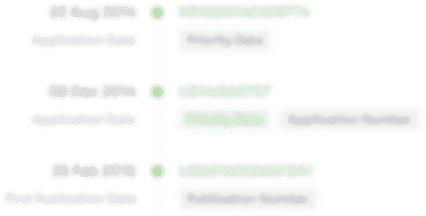
- R&D Engineer
- R&D Manager
- IP Professional
- Industry Leading Data Capabilities
- Powerful AI technology
- Patent DNA Extraction
Browse by: Latest US Patents, China's latest patents, Technical Efficacy Thesaurus, Application Domain, Technology Topic, Popular Technical Reports.
© 2024 PatSnap. All rights reserved.Legal|Privacy policy|Modern Slavery Act Transparency Statement|Sitemap|About US| Contact US: help@patsnap.com