Facial expression recognition method, convolutional neural network model training method, devices and electronic apparatus
A technology of convolutional neural network and expression recognition, which is applied in the field of convolutional neural network model training methods, devices and electronic equipment, and can solve the problems of long training time, high cost of expression recognition, scattered and complicated training process, etc.
- Summary
- Abstract
- Description
- Claims
- Application Information
AI Technical Summary
Problems solved by technology
Method used
Image
Examples
Embodiment 1
[0069] refer to figure 1 , shows a flowchart of steps of an expression recognition method according to Embodiment 1 of the present invention.
[0070] The facial expression recognition method of the present embodiment comprises the following steps:
[0071] Step S102: Using the convolutional layer part of the convolutional neural network model and the acquired face key points in the face image to be detected, perform facial expression feature extraction on the face image to be detected to obtain a face expression feature map.
[0072] The trained convolutional neural network model has the function of facial expression recognition, which at least includes an input layer part, a convolutional layer part, a pooling layer part, a fully connected layer part, etc. Among them, the input layer part is used to input the image; the convolution layer part performs feature extraction; the pooling layer part performs pooling processing on the processing results of the convolution layer part...
Embodiment 2
[0083] refer to figure 2 , shows a flowchart of steps of an expression recognition method according to Embodiment 2 of the present invention.
[0084] In this embodiment, a convolutional neural network model with a facial expression recognition function is trained first, and then facial expression recognition of images is performed based on the model. However, those skilled in the art should understand that in actual use, the convolutional neural network model trained by a third party can also be used for facial expression recognition.
[0085] The facial expression recognition method of the present embodiment comprises the following steps:
[0086] Step S202: Obtain sample images for training, and use the sample images to train a convolutional neural network model.
[0087] Wherein, the sample image may be a static image, or a sample image of a sequence of video frames. The sample image contains the information of the key points of the human face and the annotation inform...
Embodiment 3
[0107] refer to image 3 , shows a flow chart of steps of an expression recognition method according to Embodiment 3 of the present invention.
[0108]This embodiment describes the facial expression recognition method of the embodiment of the present invention in the form of a specific example. The facial expression recognition method of this embodiment includes both a convolutional neural network model training part and a facial expression recognition part using the trained convolutional neural network model.
[0109] The facial expression recognition method of the present embodiment comprises the following steps:
[0110] Step S302: Collect facial expression images and perform expression labeling to form a sample image set to be trained.
[0111] For example, ten expressions were manually marked: angry, calm, confused, disgusted, happy, sad, scared, surprised, squinting, and screaming.
[0112] Step S304: Use the face detection algorithm to detect the faces and their key ...
PUM
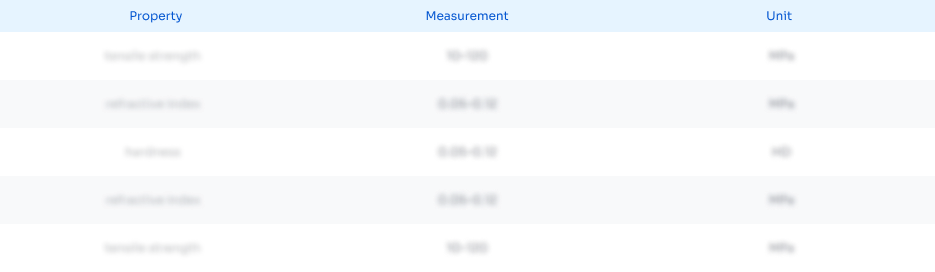
Abstract
Description
Claims
Application Information
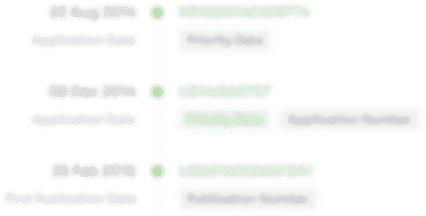
- R&D Engineer
- R&D Manager
- IP Professional
- Industry Leading Data Capabilities
- Powerful AI technology
- Patent DNA Extraction
Browse by: Latest US Patents, China's latest patents, Technical Efficacy Thesaurus, Application Domain, Technology Topic, Popular Technical Reports.
© 2024 PatSnap. All rights reserved.Legal|Privacy policy|Modern Slavery Act Transparency Statement|Sitemap|About US| Contact US: help@patsnap.com