Cyanobacterial bloom prediction method based on multi-factor time sequence-random depth confidence network model
A deep belief network and cyanobacteria bloom technology, applied in the field of water bloom prediction, can solve the problems of low prediction accuracy of water bloom and deal with highly nonlinear systems, etc., to facilitate the processing of timing problems, improve prediction accuracy, and reduce sample usage Effect
- Summary
- Abstract
- Description
- Claims
- Application Information
AI Technical Summary
Problems solved by technology
Method used
Image
Examples
Embodiment 1
[0087]Taking the chlorophyll-a concentration, influencing factor PH value, ammonia nitrogen and water temperature data in the Taihu Lake Basin in Jiangsu Province as an example, the method proposed by the present invention is used to predict cyanobacterial blooms. Taking the observation data of Taihu Lake in 2008 as an example, after data screening and normalization processing, a total of 766 samples of chlorophyll concentration data and three samples of influencing factors from July to September were selected, as follows: Figure 3 ~ Figure 6 As shown, each influencing factor consists of 764 samples, which are divided into two groups. The first set of sample data is composed of 508 samples of chlorophyll concentration and the data of influencing factors PH value, ammonia nitrogen and water temperature, and each influencing factor data is composed of 507 samples. The second set of sample data consists of 258 samples of chlorophyll concentration and the data of influencing fact...
PUM
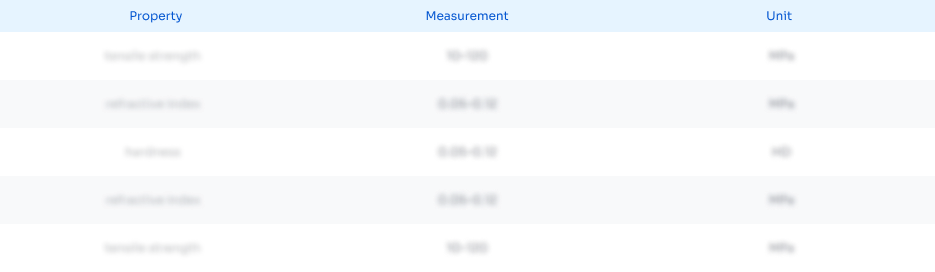
Abstract
Description
Claims
Application Information
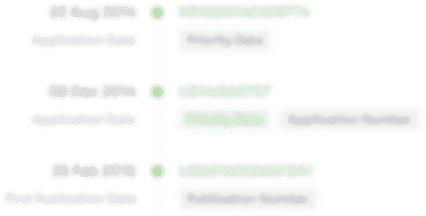
- R&D Engineer
- R&D Manager
- IP Professional
- Industry Leading Data Capabilities
- Powerful AI technology
- Patent DNA Extraction
Browse by: Latest US Patents, China's latest patents, Technical Efficacy Thesaurus, Application Domain, Technology Topic, Popular Technical Reports.
© 2024 PatSnap. All rights reserved.Legal|Privacy policy|Modern Slavery Act Transparency Statement|Sitemap|About US| Contact US: help@patsnap.com