Deep learning characteristic generalization method based on latent variable model
A deep learning and latent variable technology, applied in the field of deep learning feature generalization based on latent variables, which can solve problems such as low image quality
- Summary
- Abstract
- Description
- Claims
- Application Information
AI Technical Summary
Problems solved by technology
Method used
Image
Examples
Embodiment Construction
[0104] The preferred embodiments of the present invention will be described in detail below in conjunction with the accompanying drawings.
[0105] A preferred data flow processing method of the present invention is as follows: Image 6 As shown, the specific implementation method is as follows:
[0106] First, the original DNN needs to be divided into two parts, DNN-1 and DNN-2, where X is the feature map output by DNN-1, and its dimension is expressed as:
[0107] x dim =F num ×Size height ×Size width (32)
[0108] f num Indicates the number of current feature maps, Size height 、Size width represent the height and width of a feature map, respectively. As mentioned in the previous section, p(z|x) is a Gaussian form with an approximate diagonal covariance structure, then the posterior probability is expressed as a parameterized Gaussian distribution:
[0109]
[0110] The mapping x→p(z|x) can choose the transformation of the following form to calculate the mean v...
PUM
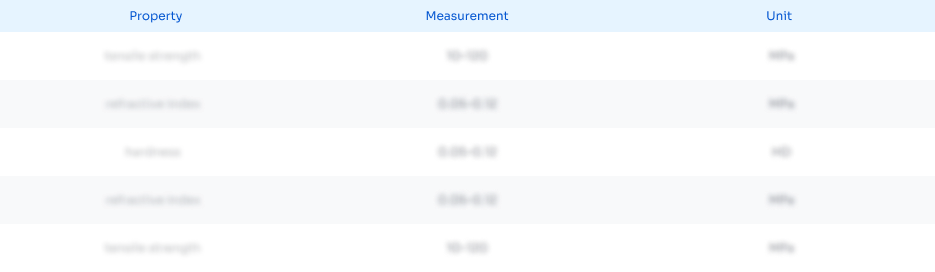
Abstract
Description
Claims
Application Information
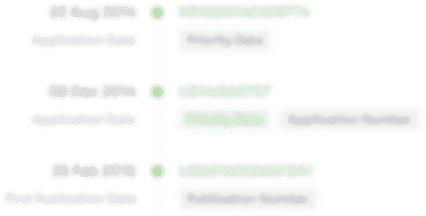
- R&D Engineer
- R&D Manager
- IP Professional
- Industry Leading Data Capabilities
- Powerful AI technology
- Patent DNA Extraction
Browse by: Latest US Patents, China's latest patents, Technical Efficacy Thesaurus, Application Domain, Technology Topic.
© 2024 PatSnap. All rights reserved.Legal|Privacy policy|Modern Slavery Act Transparency Statement|Sitemap