Self-adaptive feature fusion-based multi-scale correlation filtering visual tracking method
A multi-scale correlation and feature fusion technology, applied in the field of visual tracking of computer vision, can solve problems such as wrong tracking information, tracking failure, tracking frame drift, etc.
- Summary
- Abstract
- Description
- Claims
- Application Information
AI Technical Summary
Problems solved by technology
Method used
Image
Examples
Embodiment Construction
[0081] Such as figure 1 The shown embodiment of the present invention discloses a multi-scale correlation filter visual tracking method for adaptive feature fusion, which specifically includes the following steps:
[0082] A multi-scale correlation filtering visual tracking method for adaptive feature fusion, comprising the following steps:
[0083] Step 1. Initialization: area around the target, ideal scale filter output standard deviation, filter regularization weight factors λ, λ1, λ2, tracking model learning factor η, weight update factor δ, scale series S, scale increment factor a, The initial setting of the response threshold T; and the Hog feature size used is the target unit of 4pixel×4pixel, and the grid unit of M×N is used to represent the size of the target candidate window image block z, which is proportional to the size of the tracking frame;
[0084] Step 2. Read the video sequence using the context-aware correlation filtering framework. The framework is divided...
PUM
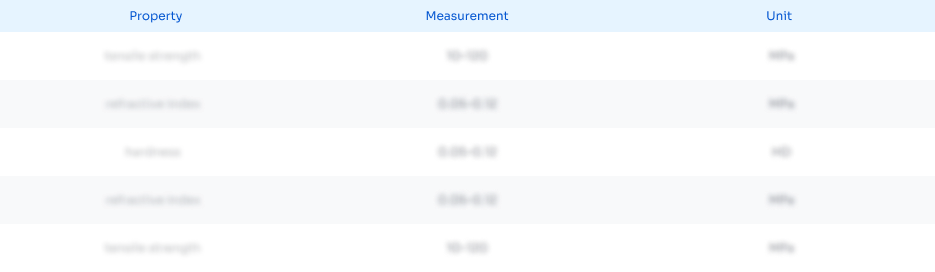
Abstract
Description
Claims
Application Information
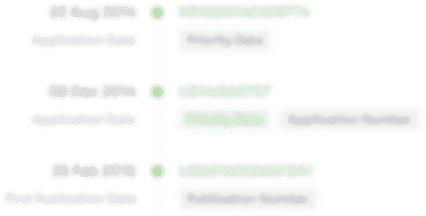
- R&D Engineer
- R&D Manager
- IP Professional
- Industry Leading Data Capabilities
- Powerful AI technology
- Patent DNA Extraction
Browse by: Latest US Patents, China's latest patents, Technical Efficacy Thesaurus, Application Domain, Technology Topic, Popular Technical Reports.
© 2024 PatSnap. All rights reserved.Legal|Privacy policy|Modern Slavery Act Transparency Statement|Sitemap|About US| Contact US: help@patsnap.com