Random weight network generalization ability improvement method and device, and computer readable storage medium
A random weight network and computer technology, applied in the field of machine learning, can solve the problems of increasing the training complexity of the integrated learning model and increasing the risk of over-fitting of the integrated learning model
- Summary
- Abstract
- Description
- Claims
- Application Information
AI Technical Summary
Problems solved by technology
Method used
Image
Examples
Embodiment Construction
[0037] In order to make the purpose, features and advantages of the present invention more obvious and understandable, the technical solutions in the embodiments of the present invention will be clearly and completely described below in conjunction with the accompanying drawings in the embodiments of the present invention. Obviously, the described The embodiments are only some of the embodiments of the present invention, but not all of them. Based on the embodiments of the present invention, all other embodiments obtained by those skilled in the art without making creative efforts belong to the protection scope of the present invention.
[0038] Such as figure 1 As shown, the method for improving the generalization ability of the random weight network provided by the embodiment of the present invention mainly includes steps S1 to S2, which will be described in detail below.
[0039] Step S1: In the initial training set Train the initial random weight network RWN on 0 , its...
PUM
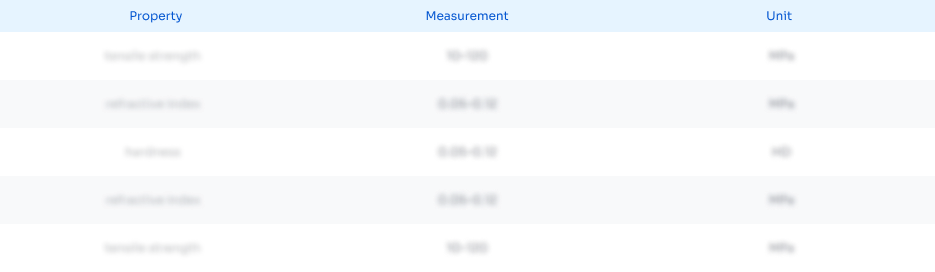
Abstract
Description
Claims
Application Information
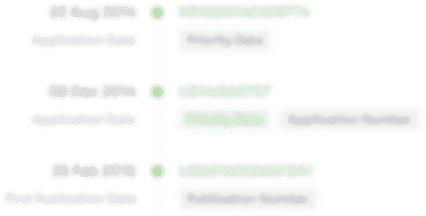
- R&D Engineer
- R&D Manager
- IP Professional
- Industry Leading Data Capabilities
- Powerful AI technology
- Patent DNA Extraction
Browse by: Latest US Patents, China's latest patents, Technical Efficacy Thesaurus, Application Domain, Technology Topic, Popular Technical Reports.
© 2024 PatSnap. All rights reserved.Legal|Privacy policy|Modern Slavery Act Transparency Statement|Sitemap|About US| Contact US: help@patsnap.com