Computer-aided model construction method based on deep learning gastric cancer pathological sections
A computer-aided pathological slicing technology, applied in computer parts, calculation, and recognition of medical/anatomical patterns, etc., can solve difficult morphological features, texture features and related structural descriptions, and it is difficult to extract distinguishable high-quality Features, general recognition accuracy and other issues, to achieve the effect of avoiding overfitting, high sensitivity, and high recognition accuracy
- Summary
- Abstract
- Description
- Claims
- Application Information
AI Technical Summary
Problems solved by technology
Method used
Image
Examples
Embodiment Construction
[0045] The present invention will be described in further detail below in conjunction with specific embodiments and with reference to the accompanying drawings.
[0046] The hardware used in the present invention is a workstation capable of deep learning. The auxiliary tool used is the deep learning training framework Keras.
[0047] The computer-aided diagnosis model construction method of gastric cancer pathological slices based on deep learning provided by the present invention mainly includes the following steps:
[0048] Step 1, build a 121-layer DenseNet model, such as Figure 8 As shown in , the backbone of the model is composed of 4 gradually deepening dense structures and 4 transition layers alternately spliced. Among them, the transition layer structure and the basic convolutional structure distribution of the dense structure are as follows: figure 2 and figure 1 . In each dense structure, before each convolution operation starts, the results of each previous c...
PUM
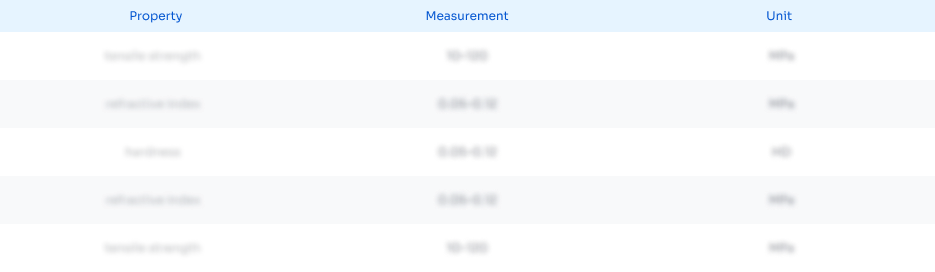
Abstract
Description
Claims
Application Information
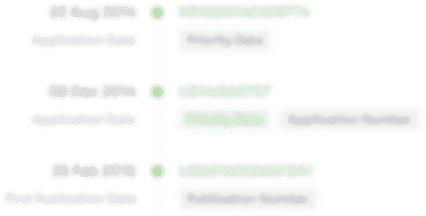
- R&D Engineer
- R&D Manager
- IP Professional
- Industry Leading Data Capabilities
- Powerful AI technology
- Patent DNA Extraction
Browse by: Latest US Patents, China's latest patents, Technical Efficacy Thesaurus, Application Domain, Technology Topic, Popular Technical Reports.
© 2024 PatSnap. All rights reserved.Legal|Privacy policy|Modern Slavery Act Transparency Statement|Sitemap|About US| Contact US: help@patsnap.com