A multi-brand chemical process soft sensor modeling method for automatically generating samples
A chemical process, automatic generation technology, applied in chemical process analysis/design, neural learning method, biological neural network model, etc., can solve the problems of high data acquisition cost and low prediction accuracy, to improve accuracy and increase samples. The amount of data, the effect of eliminating high costs and long-term data collection
- Summary
- Abstract
- Description
- Claims
- Application Information
AI Technical Summary
Problems solved by technology
Method used
Image
Examples
example
[0109] Example: A multi-brand chemical process soft-sensing modeling method that automatically generates samples. The process is as follows:
[0110] (1) Collect and divide data sets of multi-brand chemical process
[0111] The first type of brand data has 72 pieces, 50 of which are divided into training sets, and 22 pieces are divided into test sets. The second kind of brand data has 211 pieces, of which 170 pieces are divided into training sets, and 41 pieces are divided into test sets.
[0112] (2) Use multi-brand chemical process data to train AGAN
[0113] Initialize the parameters of the network, set the batch training volume (batch size) to 30, input the Gaussian distribution of noise to [0,1], and use all the training sets to train AGAN, iterate the network weights until the loss function converges, and the discriminator Balanced with the generator.
[0114] (3) Use AGAN to automatically generate multi-brand chemical process data, and build a new training set to tra...
PUM
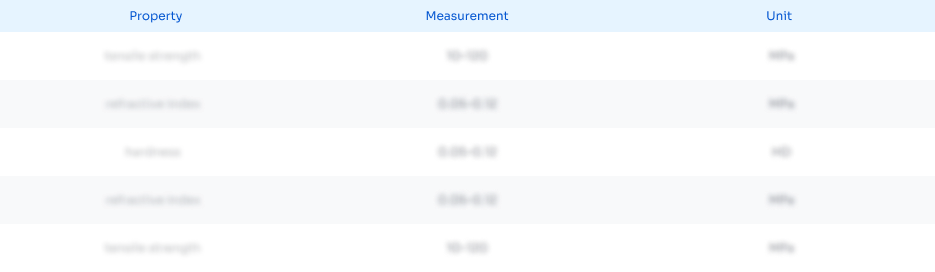
Abstract
Description
Claims
Application Information
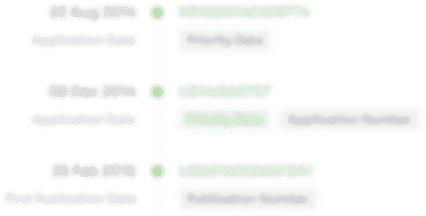
- R&D
- Intellectual Property
- Life Sciences
- Materials
- Tech Scout
- Unparalleled Data Quality
- Higher Quality Content
- 60% Fewer Hallucinations
Browse by: Latest US Patents, China's latest patents, Technical Efficacy Thesaurus, Application Domain, Technology Topic, Popular Technical Reports.
© 2025 PatSnap. All rights reserved.Legal|Privacy policy|Modern Slavery Act Transparency Statement|Sitemap|About US| Contact US: help@patsnap.com