Pipeline leakage signal identification method based on improved ELMD multi-scale entropy
A multi-scale entropy and leakage signal technology, applied in the field of ELMD multi-scale entropy pipeline leakage signal identification, can solve the problems of unsatisfactory detection effect and difficulty in obtaining pipe pressure data
- Summary
- Abstract
- Description
- Claims
- Application Information
AI Technical Summary
Problems solved by technology
Method used
Image
Examples
Embodiment Construction
[0076] The present invention will be described in detail in conjunction with accompanying drawing now. This figure is a simplified schematic diagram only illustrating the basic structure of the present invention in a schematic manner, so it only shows the components relevant to the present invention.
[0077] A pipeline leakage signal recognition method based on improved ELMD multi-scale entropy of the present invention, such as figure 1 It is a specific flow chart of the present invention. The overall local mean decomposition algorithm, multi-scale entropy, and BP neural network are comprehensively applied. The specific steps of this method are as follows:
[0078] Such as figure 2 As shown, the simulated pipe length in this experiment is 42m, the pipe material is steel, the pipe specification is DN90, the medium is compressed air, and the medium in the pipe is in a flowing state. No. 1 upstream sensor is placed at 0m, and a leak hole with a leak diameter of 1mm is locate...
PUM
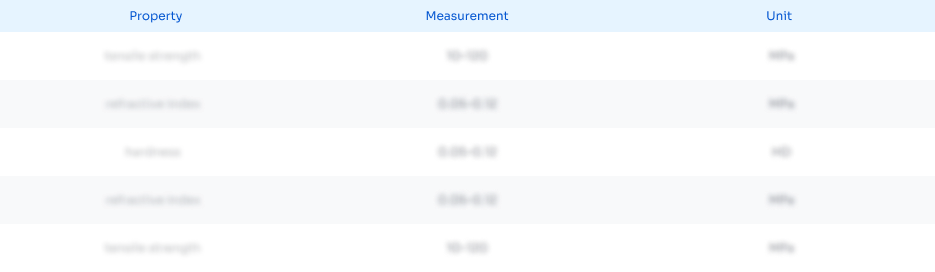
Abstract
Description
Claims
Application Information
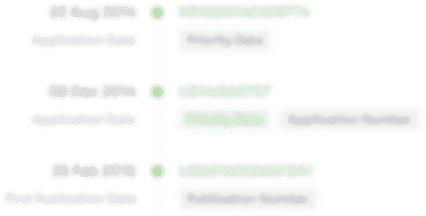
- R&D
- Intellectual Property
- Life Sciences
- Materials
- Tech Scout
- Unparalleled Data Quality
- Higher Quality Content
- 60% Fewer Hallucinations
Browse by: Latest US Patents, China's latest patents, Technical Efficacy Thesaurus, Application Domain, Technology Topic, Popular Technical Reports.
© 2025 PatSnap. All rights reserved.Legal|Privacy policy|Modern Slavery Act Transparency Statement|Sitemap|About US| Contact US: help@patsnap.com