Wheat drought recognition method based on image depth learning
An identification method and drought technology, applied in the field of wheat abiotic stress identification, can solve the problems of small coverage, time-consuming and labor-intensive, and low accuracy of drought monitoring, and achieve the effect of high accuracy.
- Summary
- Abstract
- Description
- Claims
- Application Information
AI Technical Summary
Problems solved by technology
Method used
Image
Examples
Embodiment Construction
[0021] The present invention is described in further detail now in conjunction with accompanying drawing.
[0022] figure 1 Be the flow chart of the present invention, figure 2 It is a deep learning network structure diagram with the highest accuracy of wheat drought identification and classification in the present invention.
[0023] The single-lens reflex camera used in this method is EOS700D, 18 million effective pixels, CMOS sensor, the actual focal length of the lens f=18-135mm, and the photographs are automatically stored in the SD card in JPG format. Fix the camera with a tripod when acquiring images. Each camera is equipped with a Magenta 282 signal receiver for automatic acquisition of wheat images. The camera is 1.5m away from the ground. During the drought control period, wheat images are acquired every 5 minutes from 6:00am-18:00pm every day . In order to ensure the diversity of the data and prevent the influence of the singleness of the background on the accur...
PUM
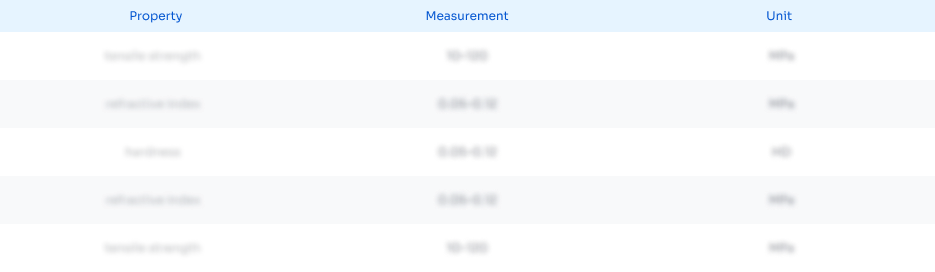
Abstract
Description
Claims
Application Information
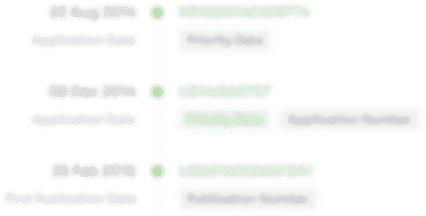
- R&D
- Intellectual Property
- Life Sciences
- Materials
- Tech Scout
- Unparalleled Data Quality
- Higher Quality Content
- 60% Fewer Hallucinations
Browse by: Latest US Patents, China's latest patents, Technical Efficacy Thesaurus, Application Domain, Technology Topic, Popular Technical Reports.
© 2025 PatSnap. All rights reserved.Legal|Privacy policy|Modern Slavery Act Transparency Statement|Sitemap|About US| Contact US: help@patsnap.com