A PM2.5 measurement method based on image features and integrated neural network
A neural network and image feature technology, applied in biological neural network models, image enhancement, image analysis, etc., can solve the problems of difficult real-time access to meteorological bureau data, real-time prediction of PM2.5 concentration, etc.
- Summary
- Abstract
- Description
- Claims
- Application Information
AI Technical Summary
Problems solved by technology
Method used
Image
Examples
Embodiment Construction
[0073] The invention obtains a kind of air fine particulate matter PM based on image feature and integrated neural network 2.5 Soft measurement method. The soft-sensing method in PM 2.5 As the output, take the image features obtained by the feature extraction method as the input, and use the integrated neural network to establish PM 2.5 soft sensor model, the PM 2.5 Make predictions.
[0074] The experimental data comes from the weather website ( http: / / www.tour-beijing.com / real_time_weather_ photo / ), collected real-time weather pictures and corresponding PM in Beijing from March 1, 2015 to April 1, 2015 on this website 2.5 Concentration data, image feature data and PM extracted from the actual pictures taken in the previous hour after removing abnormal and missing data 2.5 The data of the next hour of concentration corresponded one by one, and a total of 150 sets of data were sorted out.
[0075] A fine particulate matter PM based on image features and integrated ne...
PUM
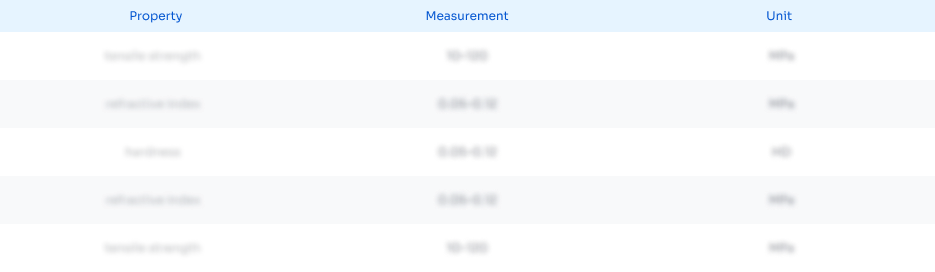
Abstract
Description
Claims
Application Information
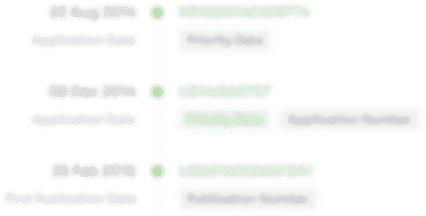
- R&D Engineer
- R&D Manager
- IP Professional
- Industry Leading Data Capabilities
- Powerful AI technology
- Patent DNA Extraction
Browse by: Latest US Patents, China's latest patents, Technical Efficacy Thesaurus, Application Domain, Technology Topic.
© 2024 PatSnap. All rights reserved.Legal|Privacy policy|Modern Slavery Act Transparency Statement|Sitemap