Target detection and recognition method for image sequence
An image sequence and target detection technology, which is applied in image analysis, image data processing, character and pattern recognition, etc., can solve the problems of inability to detect with high precision, image quality degradation, and low target detection accuracy
- Summary
- Abstract
- Description
- Claims
- Application Information
AI Technical Summary
Problems solved by technology
Method used
Image
Examples
Embodiment Construction
[0027] On the basis of the previous research, inspired by the multi-obstacle detection of autonomous driving, the single-image target detection algorithm represented by Faster R-CNN is extended to detect on video time-series images. The model architecture is as figure 2 As shown, the bottom layer is the image of each frame of the video. These images are respectively input into the shared convolutional network, and after calculating the convolutional feature map (Feature Map) of each frame, the convolutional feature map (Feature Map) of the current frame is then merged by the fusion algorithm ( Feature Map) and the convolutional feature map (Feature Map) of the front and rear multi-frames. Input the generated fused convolutional feature map (Feature Map) into the Region Proposal Network (Region Proposal Network), and the RPN (Region Proposal Network) network uses a sliding window to slide on the fused convolutional feature map (Feature Map) to generate a region recommendation ...
PUM
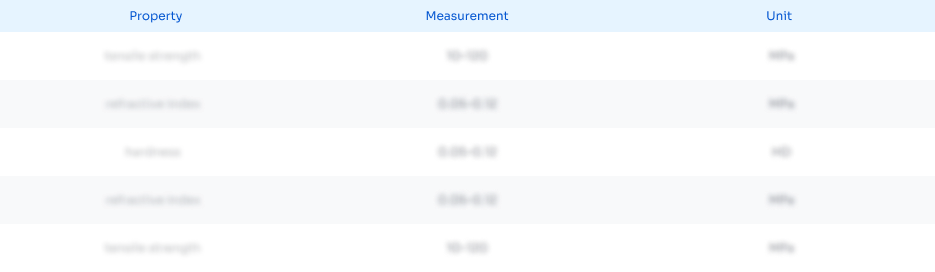
Abstract
Description
Claims
Application Information
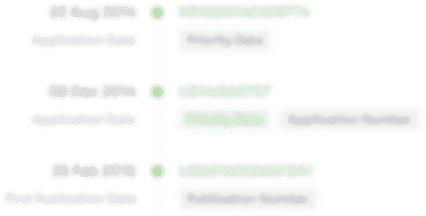
- Generate Ideas
- Intellectual Property
- Life Sciences
- Materials
- Tech Scout
- Unparalleled Data Quality
- Higher Quality Content
- 60% Fewer Hallucinations
Browse by: Latest US Patents, China's latest patents, Technical Efficacy Thesaurus, Application Domain, Technology Topic, Popular Technical Reports.
© 2025 PatSnap. All rights reserved.Legal|Privacy policy|Modern Slavery Act Transparency Statement|Sitemap|About US| Contact US: help@patsnap.com