A neural network approach to DOA estimation with array errors
A neural network and array error technology, applied in the field of signal processing, can solve problems such as high algorithm complexity, performance degradation, and variation
- Summary
- Abstract
- Description
- Claims
- Application Information
AI Technical Summary
Problems solved by technology
Method used
Image
Examples
Embodiment Construction
[0043] In order to make the above and other objects, features and advantages of the present invention more apparent, the following specifically cites the embodiments of the present invention, together with the accompanying drawings, for a detailed description as follows.
[0044] figure 1 Shown is a schematic diagram of an acoustic pressure sensor array according to an embodiment of the present invention. The ideal array of the present invention is a uniform linear array composed of N sound pressure sensor array elements arranged at equal intervals on the x-axis, and the array element interval is d x , d x ≤0.5λ min ,λ min is the minimum wavelength of the incident signal, but the actual array has position errors due to natural or artificial reasons, such as figure 1 shown.
[0045] refer to figure 2 , the steps of the neural network angle-of-arrival estimation method with array errors in the present invention are as follows: the sound pressure sensor linear array receiv...
PUM
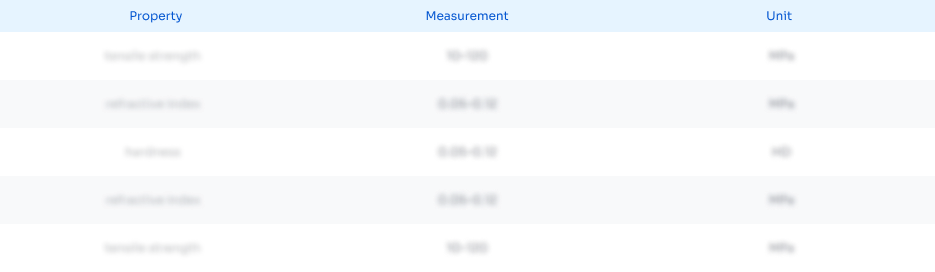
Abstract
Description
Claims
Application Information
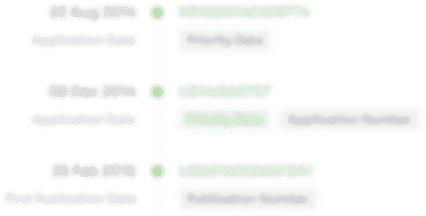
- R&D Engineer
- R&D Manager
- IP Professional
- Industry Leading Data Capabilities
- Powerful AI technology
- Patent DNA Extraction
Browse by: Latest US Patents, China's latest patents, Technical Efficacy Thesaurus, Application Domain, Technology Topic, Popular Technical Reports.
© 2024 PatSnap. All rights reserved.Legal|Privacy policy|Modern Slavery Act Transparency Statement|Sitemap|About US| Contact US: help@patsnap.com