Multi-to-multi speech conversion method based on text encoder under non-parallel text conditions
A voice conversion and encoder technology, applied in voice analysis, voice recognition, voice synthesis, etc., can solve the problems of difficult implementation, difficult to determine the number of GMM clusters, and low quality, so as to improve voice quality and similarity, and improve general Sexuality and practicality, the effect of high-quality voice conversion
- Summary
- Abstract
- Description
- Claims
- Application Information
AI Technical Summary
Problems solved by technology
Method used
Image
Examples
Embodiment Construction
[0042] Such as figure 1 As shown, the high-quality speech conversion method of the present invention is divided into two parts: the training part is used to obtain the required model parameters and conversion functions for speech conversion, and the conversion part is used to realize the conversion from the source speaker's speech to the target speaker's speech convert.
[0043] The implementation steps of the training phase are:
[0044]1.1) Obtain the training corpus of non-parallel text, the training corpus is the corpus of multiple speakers, including the source speaker and the target speaker. The training corpus is taken from the VCC2018 speech corpus, and the non-parallel text training corpus of 4 male and 4 female speakers in the corpus is selected, and each speaker has 81 sentence corpus. The corpus also contains the semantic content of each sentence in the training corpus. The training corpus of source speaker and target speaker can be either parallel text or non-p...
PUM
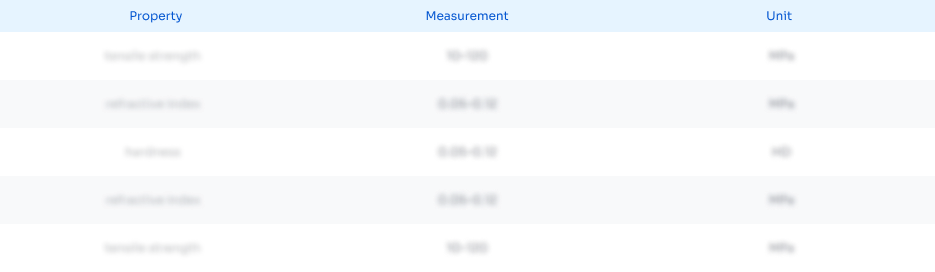
Abstract
Description
Claims
Application Information
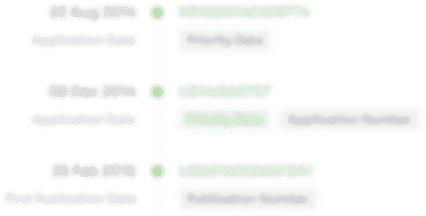
- R&D
- Intellectual Property
- Life Sciences
- Materials
- Tech Scout
- Unparalleled Data Quality
- Higher Quality Content
- 60% Fewer Hallucinations
Browse by: Latest US Patents, China's latest patents, Technical Efficacy Thesaurus, Application Domain, Technology Topic, Popular Technical Reports.
© 2025 PatSnap. All rights reserved.Legal|Privacy policy|Modern Slavery Act Transparency Statement|Sitemap|About US| Contact US: help@patsnap.com