Intelligent control method for vertical recovery of carrier rockets based on deep reinforcement learning
A launch vehicle, reinforcement learning technology, applied in attitude control, adaptive control, general control system and other directions, can solve the problems of launch vehicle orbit planning and control dependence, loss and so on
- Summary
- Abstract
- Description
- Claims
- Application Information
AI Technical Summary
Problems solved by technology
Method used
Image
Examples
Embodiment Construction
[0110] The invention proposes an intelligent control method for vertical recovery of a launch vehicle based on deep reinforcement learning, and studies a method for realizing autonomous intelligent control of a launch vehicle. The main research is to solve the problem of vertical recovery attitude control and trajectory planning of launch vehicle by using intelligent control.
[0111] The present invention first establishes the vertical recovery simulation model of the launch vehicle, and establishes the corresponding Markov decision-making process, including the state space, action space, state transition equation, and reward function, and adopts a deep reinforcement learning algorithm based on the strategy gradient, and at the same time learns from Alphago's " Decision-making network + valuation network" design idea, design the decision-making network of spacecraft and the valuation network for evaluating decision-making behavior. The decision-making network guides the space...
PUM
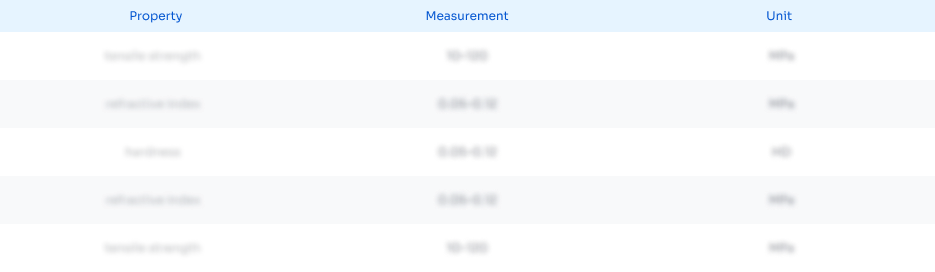
Abstract
Description
Claims
Application Information
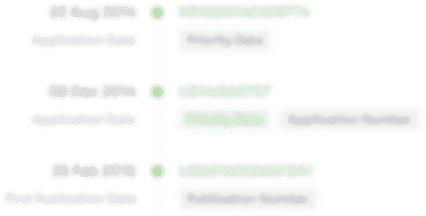
- R&D Engineer
- R&D Manager
- IP Professional
- Industry Leading Data Capabilities
- Powerful AI technology
- Patent DNA Extraction
Browse by: Latest US Patents, China's latest patents, Technical Efficacy Thesaurus, Application Domain, Technology Topic, Popular Technical Reports.
© 2024 PatSnap. All rights reserved.Legal|Privacy policy|Modern Slavery Act Transparency Statement|Sitemap|About US| Contact US: help@patsnap.com