A medical image segmentation method based on a one-way multi-task convolution neural network
A technology of convolutional neural network and medical image, which is applied in the field of medical image segmentation based on single-pass multi-task convolutional neural network, can solve the problems of complex model structure, very high hardware configuration requirements, and huge parameter volume, so as to improve the complexity of the system. degree, alleviate the problem of category imbalance, and reduce the effect of system complexity
- Summary
- Abstract
- Description
- Claims
- Application Information
AI Technical Summary
Problems solved by technology
Method used
Image
Examples
Embodiment
[0032] In order to describe the content of this embodiment conveniently, first, a brief description of the English abbreviations and technical terms in this embodiment is given:
[0033] Tissue classification and segmentation tasks: In the medical field, there are four classifications for the variant tissue of the brain region, namely: edematous tissue, necrotic tissue, non-enhanced tissue, and enhanced tissue; these four types of tissue are classified into Three overlapping areas, including: full tissue, tissue core, and enhanced tissue.
[0034] Flair sequence: One of the sequences of magnetic resonance images, commonly known as pressurized water images. In this sequence, the gray matter is hyperintense (the image appears "brighter") and the white matter is hypointense (the image appears "darker"), and these properties are similar to T2WI. But on the pressurized water image, the cerebrospinal fluid is low signal, that is, black.
[0035] Ground-truth: In foreign scientific...
PUM
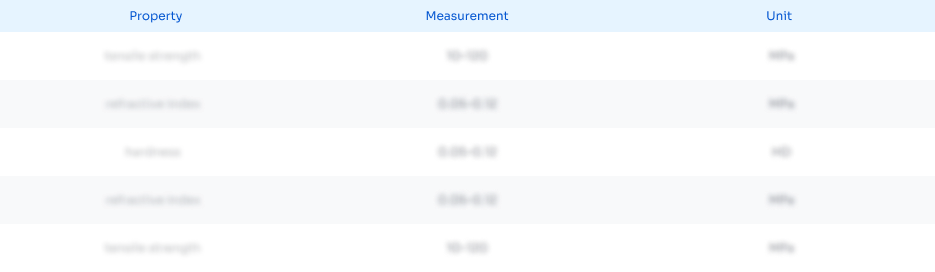
Abstract
Description
Claims
Application Information
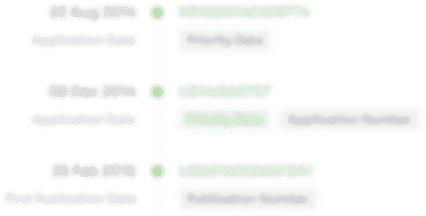
- R&D
- Intellectual Property
- Life Sciences
- Materials
- Tech Scout
- Unparalleled Data Quality
- Higher Quality Content
- 60% Fewer Hallucinations
Browse by: Latest US Patents, China's latest patents, Technical Efficacy Thesaurus, Application Domain, Technology Topic, Popular Technical Reports.
© 2025 PatSnap. All rights reserved.Legal|Privacy policy|Modern Slavery Act Transparency Statement|Sitemap|About US| Contact US: help@patsnap.com