Lane changing model parameter optimization method based on mixed Gaussian-hidden Markov model
An optimization method and model parameter technology, applied in two-dimensional position/course control, vehicle position/route/height control, non-electric variable control, etc., can solve problems such as not being the best and unreasonable model structure, and achieve good results Accuracy, the effect of improving accuracy
- Summary
- Abstract
- Description
- Claims
- Application Information
AI Technical Summary
Problems solved by technology
Method used
Image
Examples
Embodiment Construction
[0034] 1. Establishment of lane-changing intention recognition model
[0035] In order to identify the current driving behavior, by analyzing the characteristics and rules of the vehicle in the lane-changing phase, the speed of the lane-changing vehicle, the inter-vehicle distance and speed difference between the lane-changing vehicle and the front and rear vehicles in the original lane, and the target vehicle and the front and rear vehicles are selected as Observing the state parameters, and selecting lane change and going straight as the implicit state parameters, a recognition model of the driver's lane change intention is established by using the observed state to obtain the implicit state. as follows figure 1 A schematic diagram of a lane-changing vehicle is shown. where G fo is the straight-line distance between the lane-changing vehicle S and the vehicle in front of the lane-changing vehicle; G ro is the straight-line distance between the lane-changing vehicle S and ...
PUM
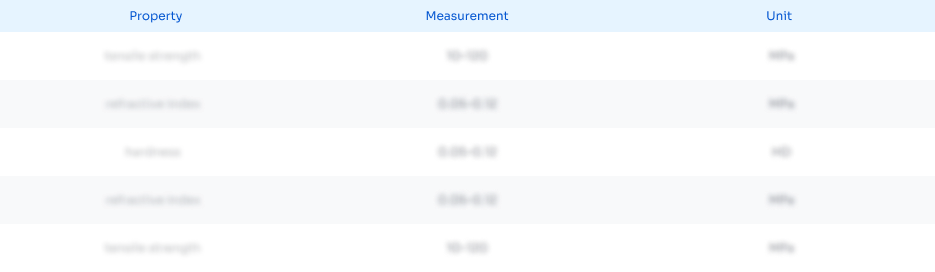
Abstract
Description
Claims
Application Information
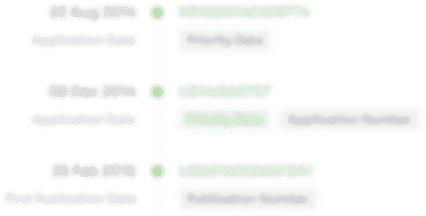
- R&D
- Intellectual Property
- Life Sciences
- Materials
- Tech Scout
- Unparalleled Data Quality
- Higher Quality Content
- 60% Fewer Hallucinations
Browse by: Latest US Patents, China's latest patents, Technical Efficacy Thesaurus, Application Domain, Technology Topic, Popular Technical Reports.
© 2025 PatSnap. All rights reserved.Legal|Privacy policy|Modern Slavery Act Transparency Statement|Sitemap|About US| Contact US: help@patsnap.com