Face aging method based on a conditional generative adversarial network
A conditional generation and network technology, applied in biological neural network models, neural learning methods, instruments, etc., can solve problems such as low image quality, inconsistent age information, and inability to maintain identity consistency, achieve high resolution, deception, etc. strong effect
- Summary
- Abstract
- Description
- Claims
- Application Information
AI Technical Summary
Problems solved by technology
Method used
Image
Examples
Embodiment 1
[0036] Such as figure 1 The flow chart of a face aging method based on conditional generative confrontation network is shown, including the following steps:
[0037] S1: Collect face data and preprocess the face data;
[0038] S11: Collect public face databases on the network, the condition is that the face database must contain age tags and identity tags, and the main databases include public databases such as FG-NET, CACD and MORPH;
[0039] S12: Perform preprocessing on the obtained image, including face detection, face cropping, and face alignment, etc., and then perform data enhancement on the data, including random cropping, and finally obtain a 224×224 standard face image.
[0040] S13: According to the existing amount of data and the needs of the model, adjust the parameters of the convolutional layer of the model once. According to experience, this method roughly divides the age of people into 16 groups, respectively {0-5,6-10 ,11-15,16-20,21-25,26-30,31-35,36-40,41...
PUM
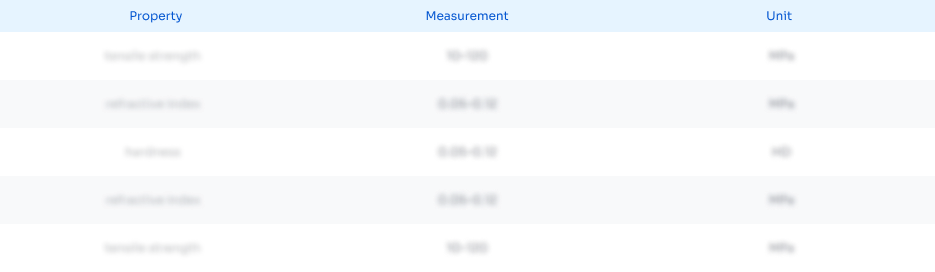
Abstract
Description
Claims
Application Information
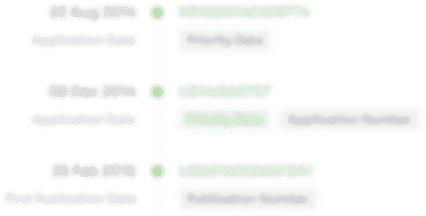
- R&D
- Intellectual Property
- Life Sciences
- Materials
- Tech Scout
- Unparalleled Data Quality
- Higher Quality Content
- 60% Fewer Hallucinations
Browse by: Latest US Patents, China's latest patents, Technical Efficacy Thesaurus, Application Domain, Technology Topic, Popular Technical Reports.
© 2025 PatSnap. All rights reserved.Legal|Privacy policy|Modern Slavery Act Transparency Statement|Sitemap|About US| Contact US: help@patsnap.com