Prediction method for PM2.5 concentration of fine particulate matters in air based on a stack selective integrated learning device
A technology of fine particulate matter and prediction method, applied in the field of machine learning, can solve problems such as difficult to control and difficult to predict PM2.5 concentration, and achieve the effect of improving accuracy
- Summary
- Abstract
- Description
- Claims
- Application Information
AI Technical Summary
Problems solved by technology
Method used
Image
Examples
Embodiment Construction
[0066] The present invention utilizes a stacked selective integrated learner to establish fine particulate matter PM in the air 2.5 Concentration prediction model, by taking the concentration of 6 pollutants and 6 meteorological indicators in the air per hour in the past 24 hours, a total of 24 groups of 12 characteristics are used as input, and the PM in the future time 2.5 Concentration is predicted. Prediction by this method has significantly improved accuracy compared with existing methods. For fine particulate matter PM in the air 2.5 Concentration prediction, with 6 pollutant concentrations and 6 meteorological indicators in the air per hour in the past 24 hours, a total of 24 groups of 12 characteristics as input;
[0067] A fine particulate matter PM in air based on stacked selective ensemble learner 2.5 The prediction method includes the following steps:
[0068] 1. Use the Android application designed by JAVA language to collect the concentration of 6 kinds of ai...
PUM
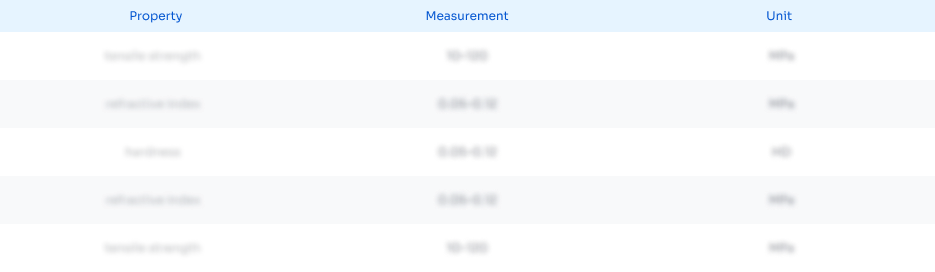
Abstract
Description
Claims
Application Information
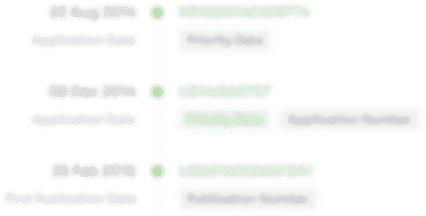
- R&D Engineer
- R&D Manager
- IP Professional
- Industry Leading Data Capabilities
- Powerful AI technology
- Patent DNA Extraction
Browse by: Latest US Patents, China's latest patents, Technical Efficacy Thesaurus, Application Domain, Technology Topic, Popular Technical Reports.
© 2024 PatSnap. All rights reserved.Legal|Privacy policy|Modern Slavery Act Transparency Statement|Sitemap|About US| Contact US: help@patsnap.com