A bilinear feature fusion fine-grained concept model and a learning method
A conceptual model and feature fusion technology, applied in the information field, can solve problems such as loss and high algorithm complexity, and achieve the effect of improving classification accuracy and high efficiency
- Summary
- Abstract
- Description
- Claims
- Application Information
AI Technical Summary
Problems solved by technology
Method used
Image
Examples
Embodiment Construction
[0047] The invention discloses a fine-grained conceptual model and a learning method for bilinear feature fusion, comprising the following steps:
[0048] Step 1, dataset preprocessing and data enhancement
[0049] Perform preprocessing and data augmentation on the dataset; specifically:
[0050] The data set is divided into training set, test set and verification set, and then the images in the training set, test set and verification set are preprocessed; the preprocessed data set is flipped horizontally to expand the data set.
[0051] In this embodiment, for the fine-grained data set, taking the FGVC Aircraft data set as an example, it is divided into a training set of 6001 samples, a verification set of 666 samples, and a test set of 3333 samples. The preprocessing process is: transform the training set data into a size of 488x488, deform the verification set and test set into a size of 448x448, and then randomly crop the verification set to a size of 448x448. The data e...
PUM
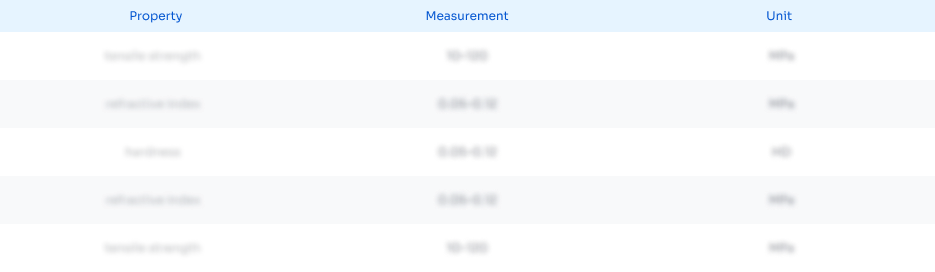
Abstract
Description
Claims
Application Information
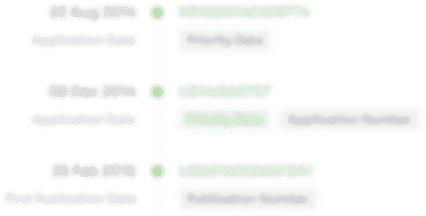
- R&D Engineer
- R&D Manager
- IP Professional
- Industry Leading Data Capabilities
- Powerful AI technology
- Patent DNA Extraction
Browse by: Latest US Patents, China's latest patents, Technical Efficacy Thesaurus, Application Domain, Technology Topic, Popular Technical Reports.
© 2024 PatSnap. All rights reserved.Legal|Privacy policy|Modern Slavery Act Transparency Statement|Sitemap|About US| Contact US: help@patsnap.com