A self-learning small sample remote sensing image classification method based on consistency constraint
A remote sensing image and classification method technology, applied in the field of remote sensing image processing, can solve problems such as waste of resources, category misjudgment, complex background of remote sensing images, etc., and achieve far-reaching practical significance and high classification accuracy
- Summary
- Abstract
- Description
- Claims
- Application Information
AI Technical Summary
Problems solved by technology
Method used
Image
Examples
Embodiment Construction
[0022] Now in conjunction with embodiment, accompanying drawing, the present invention will be further described:
[0023] Computer hardware environment for implementation: Intel Xeon E5-2683 v3@2.00GHz 14-core CPU processor, 128GB memory, equipped with GeForce GTX TITAN Xp GPU. The running software environment is: Linux16.04 64-bit operating system. We have realized the method that the present invention proposes with Matlab R2017a and python2.7 software. The remote sensing images used for training and testing in the experiment come from NWPU45: https: / / 1drv.ms / u / s! AmgKYzARBl5ca3HNaHIlzp_IXjs, such as figure 2 in the image section.
[0024] The present invention is specifically implemented as follows:
[0025] A self-learning small-sample remote sensing image classification method based on consistency constraints, characterized by the following steps:
[0026] Step 1: Perform data augmentation operations on the labeled training sample set and the unlabeled training samp...
PUM
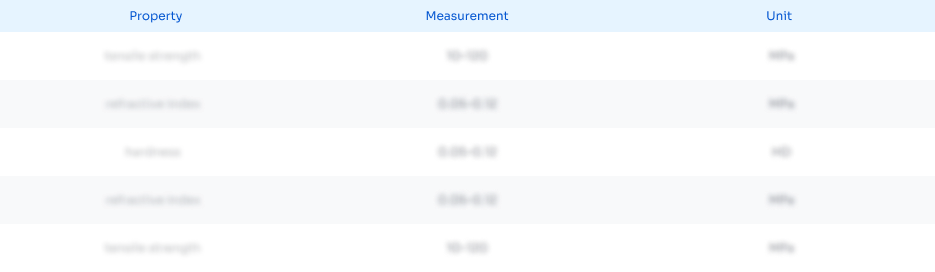
Abstract
Description
Claims
Application Information
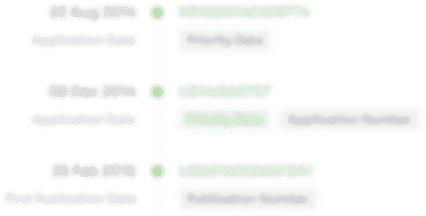
- R&D Engineer
- R&D Manager
- IP Professional
- Industry Leading Data Capabilities
- Powerful AI technology
- Patent DNA Extraction
Browse by: Latest US Patents, China's latest patents, Technical Efficacy Thesaurus, Application Domain, Technology Topic, Popular Technical Reports.
© 2024 PatSnap. All rights reserved.Legal|Privacy policy|Modern Slavery Act Transparency Statement|Sitemap|About US| Contact US: help@patsnap.com