voltage sag disturbance classification method based on LSTM
A technology of voltage sag and classification method, applied in instruments, biological neural network models, character and pattern recognition, etc., can solve the problem of missing signal features, low accuracy of signal classification and recognition, and complicated and cumbersome manual feature extraction steps for sag signals. and other problems, to achieve high complexity, good anti-noise performance and generalization ability, and improve the classification accuracy.
- Summary
- Abstract
- Description
- Claims
- Application Information
AI Technical Summary
Problems solved by technology
Method used
Image
Examples
Embodiment
[0033] like figure 1 As shown, the LSTM-based voltage sag disturbance classification method of this embodiment builds a four-layer deep learning network model containing a data processing layer, a 2-layer LSTM layer, a fully connected layer, and a classification layer, wherein the data processing layer is also the entire model The input layer, the classification layer is also the result output layer.
[0034] like figure 2 As shown, the training process of the model in this embodiment includes two steps of unsupervised pre-training and model fine-tuning, wherein the unsupervised pre-training process is used to determine the hyperparameters such as the number of neurons and the learning rate of each layer of the entire model, and the model fine-tuning is On the basis of pre-training, fine-tuning of parameters such as the weight of the model is performed, and the LSTM-based voltage sag disturbance classification model of the present invention is finally obtained through these ...
PUM
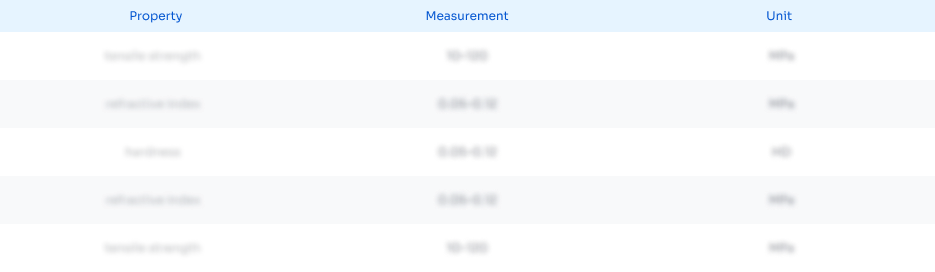
Abstract
Description
Claims
Application Information
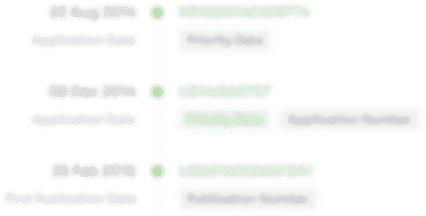
- Generate Ideas
- Intellectual Property
- Life Sciences
- Materials
- Tech Scout
- Unparalleled Data Quality
- Higher Quality Content
- 60% Fewer Hallucinations
Browse by: Latest US Patents, China's latest patents, Technical Efficacy Thesaurus, Application Domain, Technology Topic, Popular Technical Reports.
© 2025 PatSnap. All rights reserved.Legal|Privacy policy|Modern Slavery Act Transparency Statement|Sitemap|About US| Contact US: help@patsnap.com