Lane-level precision automatic driving structured data analysis method
A structured data, autonomous driving technology, applied in neural learning methods, neural architectures, instruments, etc., can solve problems such as low accuracy of lane marking extraction, unclear boundary attributes, road wear and other factors, etc. Achieve the effect of increasing lane boundary branches, strengthening feature extraction capabilities, and improving accuracy
- Summary
- Abstract
- Description
- Claims
- Application Information
AI Technical Summary
Problems solved by technology
Method used
Image
Examples
Embodiment
[0053] In this embodiment, a lane-level precision automatic driving structured data analysis method, such as figure 1 shown, including the following steps:
[0054] S1: Establish a multi-task deep convolutional neural network model based on shared shallow convolutional features;
[0055] S2: Offline training of the multi-task deep convolutional neural network model;
[0056] S3: Transplant the front-end platform of the trained multi-task deep convolutional neural network model;
[0057] S4: Use the transplanted model to analyze the road driving scene and perform output post-processing.
[0058] The multi-task deep convolutional neural network model, such as figure 2 As shown, it includes an input layer 1, a shared feature encoding layer 2 and a lane structured data output decoding layer. The shared feature encoding layer is composed of a cascaded conv+relu+BN combination, and the lane structured data output decoding layer includes a Driving area branch 3, lane boundary br...
PUM
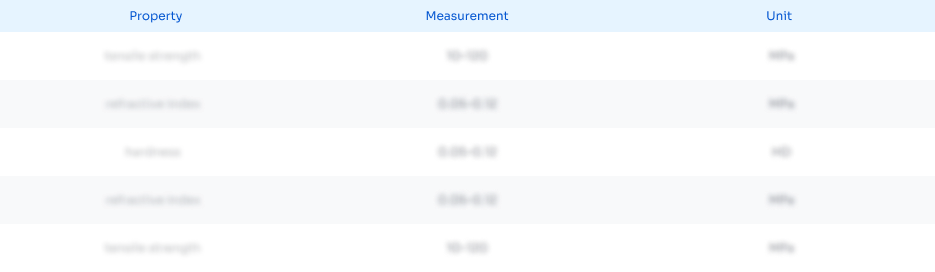
Abstract
Description
Claims
Application Information
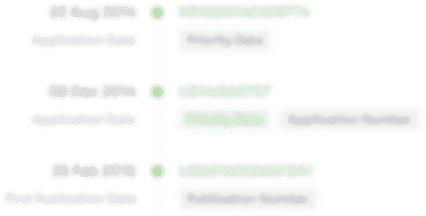
- R&D Engineer
- R&D Manager
- IP Professional
- Industry Leading Data Capabilities
- Powerful AI technology
- Patent DNA Extraction
Browse by: Latest US Patents, China's latest patents, Technical Efficacy Thesaurus, Application Domain, Technology Topic, Popular Technical Reports.
© 2024 PatSnap. All rights reserved.Legal|Privacy policy|Modern Slavery Act Transparency Statement|Sitemap|About US| Contact US: help@patsnap.com