Real image denoising method based on generative adversarial network noise modeling
A real image and network noise technology, applied in image enhancement, image data processing, instrumentation, etc., can solve problems such as difficult explicit modeling and complex noise distribution, so as to improve robustness, reduce computing burden, and improve denoising performance Effect
- Summary
- Abstract
- Description
- Claims
- Application Information
AI Technical Summary
Problems solved by technology
Method used
Image
Examples
Embodiment Construction
[0036] specific implementation plan
[0037] The specific implementation of the real image denoising method based on generative confrontation network noise modeling provided by the present invention will be described in detail below in conjunction with the accompanying drawings:
[0038] attached figure 1 An overall block diagram of a technical solution of a real image denoising method based on generative adversarial network noise modeling provided by an embodiment of the present invention. The present invention adopts a distributed network structure, including two modules of generative confrontation network noise modeling and denoising network.
[0039] The noise modeling network structure in the embodiment of the present invention is as follows figure 2 shown. Specific steps are as follows:
[0040] Step S1.1: Noise block extraction. Given a pair of data (x,y), first in the clear image y, through the sliding step S p Select the image block y(p i ), and then in y(p i...
PUM
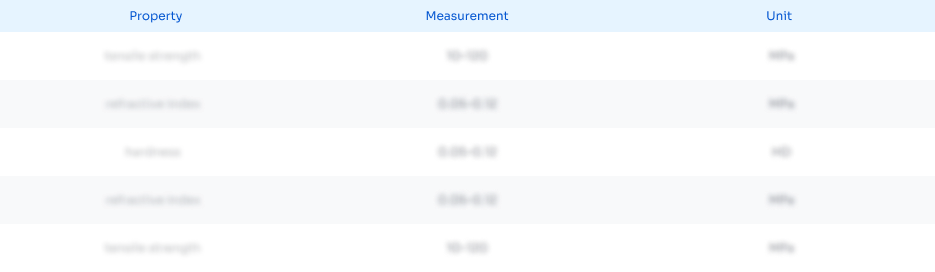
Abstract
Description
Claims
Application Information
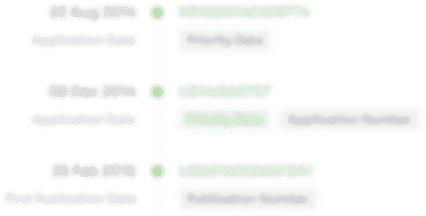
- R&D Engineer
- R&D Manager
- IP Professional
- Industry Leading Data Capabilities
- Powerful AI technology
- Patent DNA Extraction
Browse by: Latest US Patents, China's latest patents, Technical Efficacy Thesaurus, Application Domain, Technology Topic, Popular Technical Reports.
© 2024 PatSnap. All rights reserved.Legal|Privacy policy|Modern Slavery Act Transparency Statement|Sitemap|About US| Contact US: help@patsnap.com