Unmanned surface ship optimal trajectory tracking control method based on reinforced learning method
A technology of reinforcement learning and trajectory tracking, applied in two-dimensional position/channel control, non-electric variable control, control/regulation system, etc. Problems such as reduced stickiness
- Summary
- Abstract
- Description
- Claims
- Application Information
AI Technical Summary
Problems solved by technology
Method used
Image
Examples
Embodiment 1
[0140] The basic principle of reinforcement learning technology is: if a certain state of the controlled system receives a "positive" reward from the environment, it is a reinforcement signal, and the trend of each subsequent action of the system will be strengthened; otherwise, the system will produce a trend of this state. weakened. Therefore, the goal of reinforcement learning is to learn a behavior policy that enables the state of the system output to obtain the maximum reward from the environment. In a standard reinforcement learning framework, it mainly has four elements, namely policy, reward and punishment feedback (reward), cost function (cost function) and environment model (model of environment).
[0141] Such as Figure 1-2 As shown, the present invention provides a kind of unmanned surface ship optimal trajectory tracking control method based on reinforcement learning method, comprises the following steps:
[0142] S1: Establish the mathematical model M1 of the un...
PUM
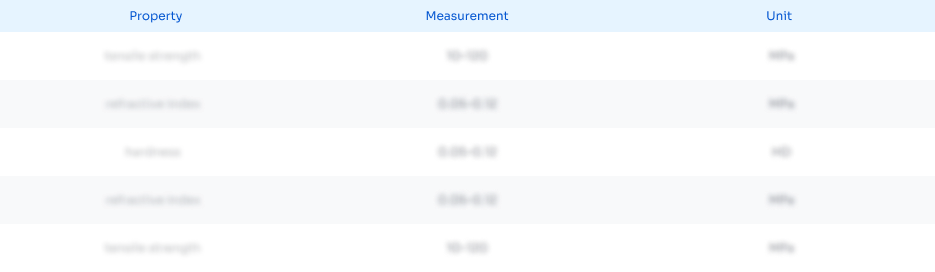
Abstract
Description
Claims
Application Information
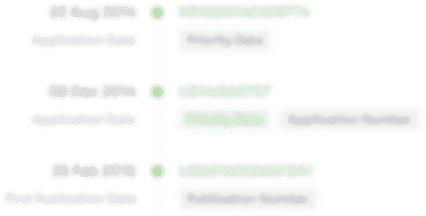
- R&D Engineer
- R&D Manager
- IP Professional
- Industry Leading Data Capabilities
- Powerful AI technology
- Patent DNA Extraction
Browse by: Latest US Patents, China's latest patents, Technical Efficacy Thesaurus, Application Domain, Technology Topic.
© 2024 PatSnap. All rights reserved.Legal|Privacy policy|Modern Slavery Act Transparency Statement|Sitemap