Incremental equipment fault diagnosis method based on knowledge distillation and hidden layer sharing
A technology for equipment failure and diagnostic methods, applied in instrumentation, electrical testing/monitoring, control/regulation systems, etc.
- Summary
- Abstract
- Description
- Claims
- Application Information
AI Technical Summary
Problems solved by technology
Method used
Image
Examples
Embodiment Construction
[0042] In order to make the objectives, technical solutions, and advantages of the embodiments of the present invention clearer, the technical solutions in the embodiments of the present invention will be described clearly and completely in conjunction with the accompanying drawings in the embodiments of the present invention. Obviously, the described embodiments It is a part of the embodiments of the present invention, not all the embodiments.
[0043] The theoretical basis of the method of the present invention:
[0044] 1. Convolutional neural network: A type of feedforward neural network that contains convolutional calculations and has a deep structure. It is one of the representative algorithms of deep learning. Because the convolutional neural network can perform translation invariant classification, it is also called "translation invariant artificial neural network".
[0045] 2. Convolutional layer: The convolutional layer is the main component of feature extraction in the co...
PUM
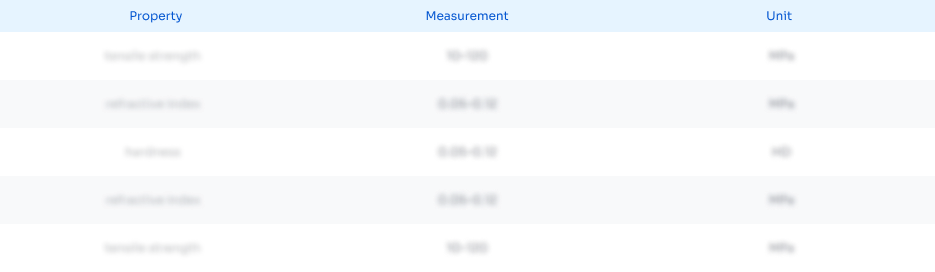
Abstract
Description
Claims
Application Information
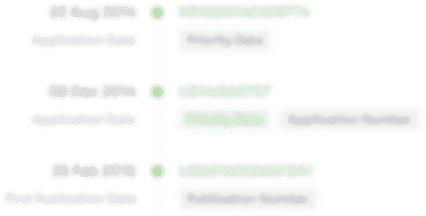
- R&D Engineer
- R&D Manager
- IP Professional
- Industry Leading Data Capabilities
- Powerful AI technology
- Patent DNA Extraction
Browse by: Latest US Patents, China's latest patents, Technical Efficacy Thesaurus, Application Domain, Technology Topic, Popular Technical Reports.
© 2024 PatSnap. All rights reserved.Legal|Privacy policy|Modern Slavery Act Transparency Statement|Sitemap|About US| Contact US: help@patsnap.com