Distribution transformer heavy overload early warning method based on load data imagination convolutional neural network
A technology of convolutional neural network and data image, applied in power system distribution network transformer load prediction, power system analysis and calculation fields, can solve problems such as inability to synthesize historical information, find high peak value, fuzzy prediction effect, etc., and achieve economic value High, innovative ideas, good versatility
- Summary
- Abstract
- Description
- Claims
- Application Information
AI Technical Summary
Problems solved by technology
Method used
Image
Examples
Embodiment 1
[0031] The distribution transformer overload warning method based on the imaged convolutional neural network of load data described in the present invention is based on the distribution transformer in a certain area under a certain power grid to perform distribution transformer overload prediction from October 2018 to November 2018, And compared with the actual heavy overload situation, the specific steps are as follows:
[0032] (1) Obtain the original instantaneous current data in a certain area from April 2018 to October 2018 in the metering automation system. The collection frequency is 15 minutes, and the 15-minute data is fused into a 96-dimensional data in days. Data, and then obtain the rated current and rated capacity data of each transformer, and calculate the load rate according to the original current data according to the standard calculation formula of China Southern Power Grid. The specific method is as follows:
[0033] Replace rated capacity with rated current...
PUM
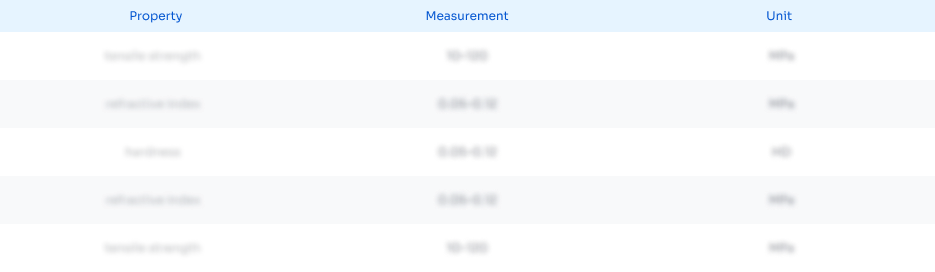
Abstract
Description
Claims
Application Information
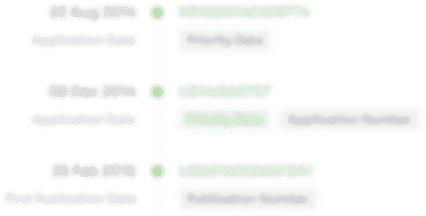
- R&D
- Intellectual Property
- Life Sciences
- Materials
- Tech Scout
- Unparalleled Data Quality
- Higher Quality Content
- 60% Fewer Hallucinations
Browse by: Latest US Patents, China's latest patents, Technical Efficacy Thesaurus, Application Domain, Technology Topic, Popular Technical Reports.
© 2025 PatSnap. All rights reserved.Legal|Privacy policy|Modern Slavery Act Transparency Statement|Sitemap|About US| Contact US: help@patsnap.com