Iterative incremental dialogue intention category identification method based on small sample
A recognition method and small sample technology, applied in the computer field, can solve the problems of slow query, manual labeling, time-consuming and labor-intensive, etc., and achieve the effect of ensuring robustness and accuracy
- Summary
- Abstract
- Description
- Claims
- Application Information
AI Technical Summary
Problems solved by technology
Method used
Image
Examples
specific Embodiment approach
[0053] The specific implementation of the iterative incremental dialogue intent recognition method based on small samples is as follows:
[0054] The first is to divide the data of telecom customer service intentions, and divide the customer service intentions into 50 categories according to the actual needs of the industry (X takes 50). The average number of important dialogue rounds is 10, and each round contains two sentences. Therefore, a customer service sample consists of about 20 sentences and an intent label. After word segmentation, each sentence has an average of about 5-10 words. Here, 1000 small sample data sets are first selected, and the 1000 data sets are divided into 10 groups, each group contains 100 data, and each group of data is divided into Da data set and Db data set again. For each set of data sets, 70% is used as the training set of a single model, and 30% is used as the verification set to evaluate the effect of the model. Until the accuracy of the mod...
PUM
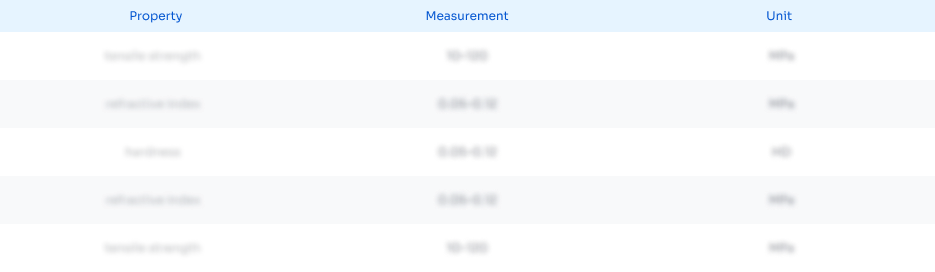
Abstract
Description
Claims
Application Information
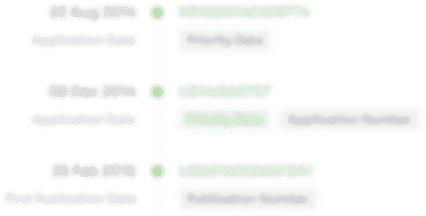
- R&D
- Intellectual Property
- Life Sciences
- Materials
- Tech Scout
- Unparalleled Data Quality
- Higher Quality Content
- 60% Fewer Hallucinations
Browse by: Latest US Patents, China's latest patents, Technical Efficacy Thesaurus, Application Domain, Technology Topic, Popular Technical Reports.
© 2025 PatSnap. All rights reserved.Legal|Privacy policy|Modern Slavery Act Transparency Statement|Sitemap|About US| Contact US: help@patsnap.com