Object Tracking Method and System Based on Triple Convolutional Network and Perceptual Interference Learning
A target tracking and convolutional network technology, applied in the field of target tracking research, can solve the problems of indistinguishable intra-class interference and inaccurate target tracking, and achieve the effect of enhancing the ability to distinguish intra-class interference, reducing drift, and improving accuracy
- Summary
- Abstract
- Description
- Claims
- Application Information
AI Technical Summary
Problems solved by technology
Method used
Image
Examples
Embodiment Construction
[0055]In order to make the objectives, technical solutions and advantages of the present invention, the present invention will be described in further detail below with reference to the accompanying drawings and examples. It should be understood that the specific embodiments described herein are merely intended to illustrate the invention and are not intended to limit the invention. Further, the technical features according to each of the various embodiments described below can be combined with each other as long as they do not constitute a collision between each other.
[0056]Such asfigure 1 As shown, a target tracking method based on triple convolutional network and perceived interference learning, by increasing the first frame branch and joining a perceptual interference learning, the network robustness can be stronger, and the accuracy of tracking is improved. Include the following steps:
[0057]Step 1: Prerequisites Target Tracking Tracking Data Set: The data set is a VID dataset. ...
PUM
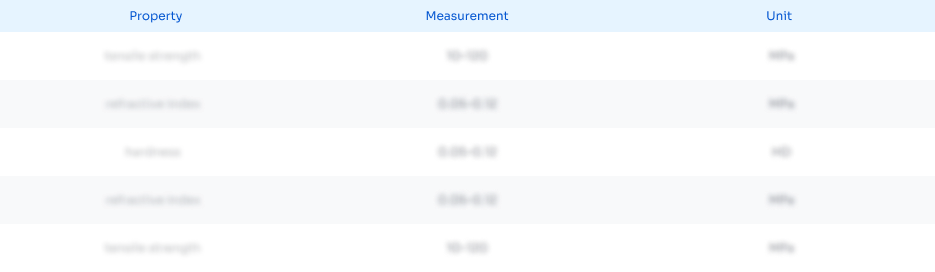
Abstract
Description
Claims
Application Information
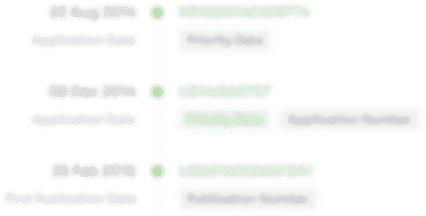
- R&D Engineer
- R&D Manager
- IP Professional
- Industry Leading Data Capabilities
- Powerful AI technology
- Patent DNA Extraction
Browse by: Latest US Patents, China's latest patents, Technical Efficacy Thesaurus, Application Domain, Technology Topic, Popular Technical Reports.
© 2024 PatSnap. All rights reserved.Legal|Privacy policy|Modern Slavery Act Transparency Statement|Sitemap|About US| Contact US: help@patsnap.com