Sea surface image semantic segmentation method based on space constraint hybrid model capable of being simplified
A mixed model and space-constrained technology, applied in the field of image processing, can solve problems such as only sky areas, sea water areas, potential obstacle areas, and errors
- Summary
- Abstract
- Description
- Claims
- Application Information
AI Technical Summary
Problems solved by technology
Method used
Image
Examples
Embodiment 1
[0073] Such as figure 1 As shown in , a semantic segmentation method for sea surface images based on a simplified spatially constrained hybrid model, the specific implementation steps are as follows:
[0074] (1) Input the color image of the sea surface to be detected;
[0075] The color image of the sea surface (with a resolution of 512×512) was obtained by the camera carried by the unmanned surface vehicle. In order to reduce the execution time of subsequent algorithms, the sea surface image is scaled to a resolution of 100×100. Such as figure 2 a shows the image of the sea surface to be detected in this embodiment, and the images used mainly include the sky, the coast, sea waves, and obstacle buoys.
[0076] (2) Assume that there are three main semantic areas of sky, coast / haze, and sea water in the sea surface image, as well as potential obstacle areas, and establish a hybrid model of spatial constraints;
[0077] It is assumed that the mixture model consists of 3 Gau...
Embodiment 2
[0106] image 3 It is a preferred embodiment of the method of the present invention under the background of no coast / haze. Its specific implementation steps are the same as those in Embodiment 1, so they are not repeated here. As can be seen from the semantic segmentation results of Embodiment 1 and Embodiment 2, the method of the present invention can automatically select whether to simplify the mixed model of space constraints according to the actual situation of the sea surface image, thereby improving the accuracy of the semantic segmentation of the sea surface image .
PUM
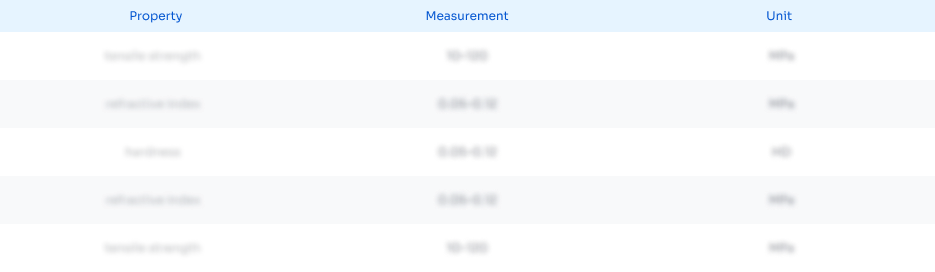
Abstract
Description
Claims
Application Information
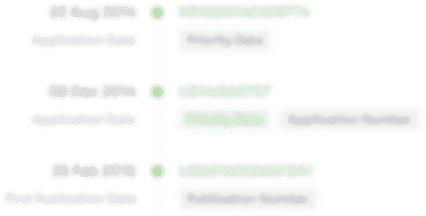
- R&D
- Intellectual Property
- Life Sciences
- Materials
- Tech Scout
- Unparalleled Data Quality
- Higher Quality Content
- 60% Fewer Hallucinations
Browse by: Latest US Patents, China's latest patents, Technical Efficacy Thesaurus, Application Domain, Technology Topic, Popular Technical Reports.
© 2025 PatSnap. All rights reserved.Legal|Privacy policy|Modern Slavery Act Transparency Statement|Sitemap|About US| Contact US: help@patsnap.com