EEG signal feature dimension reduction method based on weighted principal component analysis
A weighted principal component and signal feature technology, applied in the field of dimensionality reduction of EEG signal features based on weighted principal component analysis, can solve problems such as different and inequal treatment
- Summary
- Abstract
- Description
- Claims
- Application Information
AI Technical Summary
Problems solved by technology
Method used
Image
Examples
Embodiment Construction
[0060] A method for dimension reduction of EEG signal features based on weighted principal component analysis of the present invention will be described in detail below with reference to the embodiments and the accompanying drawings.
[0061] A method for dimensionality reduction of EEG signal features based on weighted principal component analysis of the invention, first removes one feature in turn, and counts the influence of different features on the fatigue state classification performance, and then normalizes the accuracy drop value of different features as the The weight of the feature, and finally the method of principal component analysis is introduced to establish the method of weighted principal component analysis to reduce the dimension of the feature.
[0062] A method for dimensionality reduction of EEG signal features based on weighted principal component analysis of the invention includes the following steps:
[0063] 1) Apply the AR model to extract m samples o...
PUM
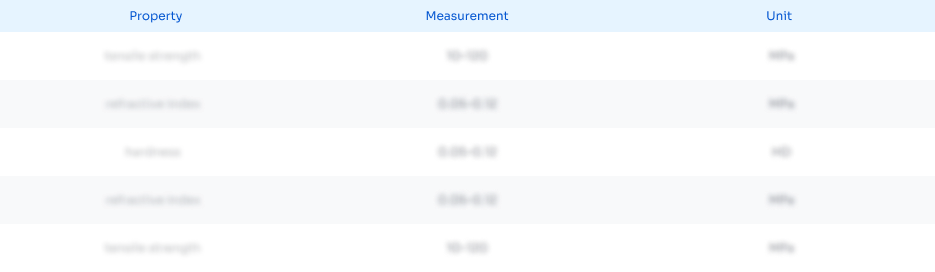
Abstract
Description
Claims
Application Information
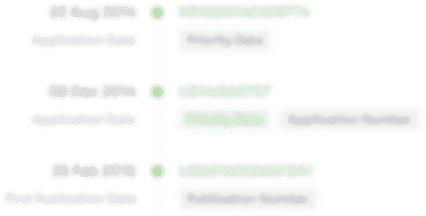
- R&D Engineer
- R&D Manager
- IP Professional
- Industry Leading Data Capabilities
- Powerful AI technology
- Patent DNA Extraction
Browse by: Latest US Patents, China's latest patents, Technical Efficacy Thesaurus, Application Domain, Technology Topic.
© 2024 PatSnap. All rights reserved.Legal|Privacy policy|Modern Slavery Act Transparency Statement|Sitemap