Method for classifying multi-label texts by using Co-Attention model based on multi-step discrimination
A text classification and multi-label technology, which is applied in text database clustering/classification, neural learning methods, biological neural network models, etc., can solve the problems of late prediction impact and failure to alleviate error accumulation, so as to improve representation ability and alleviate errors Accumulate problems and optimize the effect of the training process
- Summary
- Abstract
- Description
- Claims
- Application Information
AI Technical Summary
Problems solved by technology
Method used
Image
Examples
Embodiment 1
[0095] A method based on a multi-step discriminant Co-Attention model for multi-label text classification, such as figure 1 shown, including the following steps:
[0096] (1) Label data preprocessing: the label sequence is divided into leading labels and to-be-predicted labels. The leading labels refer to the labels that have been predicted, and the to-be-predicted labels refer to unpredicted new labels. Information fusion is performed on the leading labels and the original text. Make it meet the multi-label classification requirements of multi-step discrimination;
[0097] (2) Training word vectors; perform word vector training through the skip-gram model in word2vec, so that each word in the original text has a corresponding feature representation in the vector space; then perform downstream tasks of the model;
[0098] (3) Text feature extraction; the original text after step (2) word vector training is input into two-way LSTM model, carries out coding operation, extracts ...
Embodiment 2
[0104] A method for multi-label text classification based on a multi-step discriminant Co-Attention model according to Embodiment 1, the difference is that in step (4), feature combinations, such as Figure 4 As shown, including mutual attention operation, difference operation, cascade operation; hidden layer state vector h output for text feature extraction N and the output sequence {w 1 ,w 2 ,...,w N} Input to the feature fusion module for mutual attention operation, difference operation and cascade operation, output sequence {w 1 ,w 2 ,...,w N} and leading tag feature sequence {l 1 , l 2 ,...,l M} After mutual attention operation, two feature vectors A with weight information are obtained respectively YS 、A SY ;A YS Represents the information corresponding to the leading label in the original text. This part of the information has no effect on predicting new labels, so we delete it, that is, in h N Delete A by differential operation based on YS , h N Get the or...
PUM
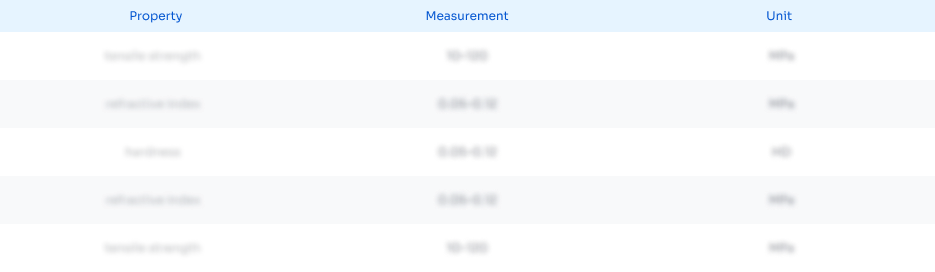
Abstract
Description
Claims
Application Information
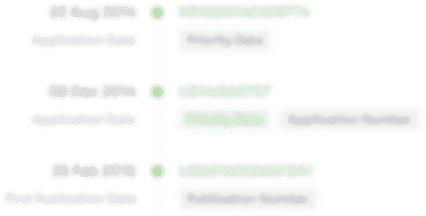
- R&D Engineer
- R&D Manager
- IP Professional
- Industry Leading Data Capabilities
- Powerful AI technology
- Patent DNA Extraction
Browse by: Latest US Patents, China's latest patents, Technical Efficacy Thesaurus, Application Domain, Technology Topic, Popular Technical Reports.
© 2024 PatSnap. All rights reserved.Legal|Privacy policy|Modern Slavery Act Transparency Statement|Sitemap|About US| Contact US: help@patsnap.com