Non-invasive load decomposition method and system
A load decomposition and non-intrusive technology, applied in the direction of AC network circuits, electrical components, circuit devices, etc., can solve the problem of low accuracy of load characteristics, achieve the effect of improving accuracy and accuracy, and increasing the receptive field
- Summary
- Abstract
- Description
- Claims
- Application Information
AI Technical Summary
Problems solved by technology
Method used
Image
Examples
Embodiment 1
[0069] This embodiment provides a non-intrusive load decomposition method, the method flow chart is as follows figure 1 shown.
[0070] S1: Obtain the total load power sequence of the target user within a period of time.
[0071] The long-term power consumption information of various electrical appliances in more than 600 households and the total power consumption of the entire household are collected, including the historical power consumption data of air conditioners, refrigerators, washing machines, dishwashers, and microwave ovens in the households, which are used for load decomposition tasks. The main reasons for choosing these types of electrical appliances are as follows: (1) The amount of supervised learning experiments on each electrical appliance in each family is huge and unnecessary, while experiments on some common and representative electrical appliances necessary. (2) Some small power consumption appliances contained in the data set are easily disturbed by noi...
Embodiment 2
[0115] This example provides a description of the residual structure used in a non-intrusive load decomposition method.
[0116] (1) Residual structure in the residual network: The residual network draws on the idea of cross-layer connection in high-speed networks. It may be difficult to simply fit an identity map H(x)=x with a forward neural network stacked with one or more hidden layers. However, if the network is designed in the form of H(x)=F(x)+x, the function can be converted into a residual function F(x)=H(x)-x, when F(x)=0, we get The mapping function H(x)=x. The residual network is such a structure that directly transmits the output of the front layer to the back layer by adding an identity map. The residual structure replaces the desired output H(x) with F(x)+x by adding an identity transformation. The two expressions have the same effect on network training, but the optimization difficulty is different. F(x)+x can pass a forward A neural network is implemented ...
Embodiment 3
[0156] This embodiment provides a non-intrusive load splitting system, including:
[0157] Data acquisition module: acquire the total load power sequence of the target user within a period of time;
[0158] Decomposition module: based on the total load power sequence of the user and the set load type, according to the relationship between the pre-trained total power sequence and the power characteristic sequence of each load type, decompose the total load power to obtain the total load power within the time period. The power characteristic sequence of each load type;
[0159] Wherein, the load type is determined by the user's usage of the household appliances;
[0160] The training process in the decomposition module includes: receptive field amplification based on identity mapping and timing.
[0161] The decomposition module includes:
[0162] Multi-user data acquisition sub-module: acquire historical multi-user load total power sequence;
[0163] Use data acquisition su...
PUM
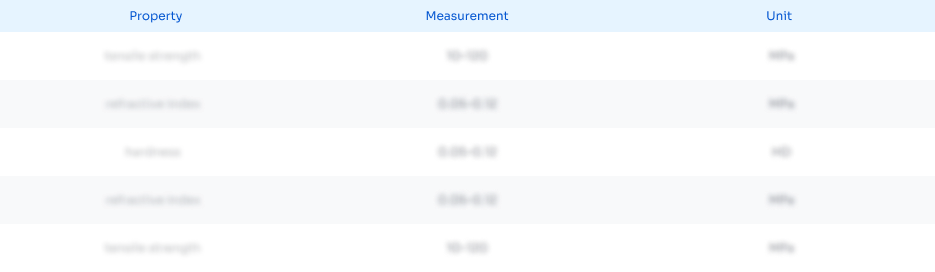
Abstract
Description
Claims
Application Information
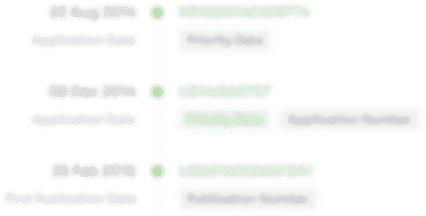
- R&D Engineer
- R&D Manager
- IP Professional
- Industry Leading Data Capabilities
- Powerful AI technology
- Patent DNA Extraction
Browse by: Latest US Patents, China's latest patents, Technical Efficacy Thesaurus, Application Domain, Technology Topic, Popular Technical Reports.
© 2024 PatSnap. All rights reserved.Legal|Privacy policy|Modern Slavery Act Transparency Statement|Sitemap|About US| Contact US: help@patsnap.com