Strip steel surface defect detection method based on deep learning
A defect detection and deep learning technology, applied in the field of deep learning-based strip steel surface defect detection, can solve problems such as high labeling cost, insufficient docking degree, mutual mixing, etc., to improve detection accuracy, reduce model scale, and reduce error rate Effect
- Summary
- Abstract
- Description
- Claims
- Application Information
AI Technical Summary
Problems solved by technology
Method used
Image
Examples
Embodiment Construction
[0041]The present invention will be described in detail below in conjunction with the accompanying drawings and specific embodiments. This embodiment is carried out on the premise of the technical solution of the present invention, and detailed implementation and specific operation process are given, but the protection scope of the present invention is not limited to the following embodiments.
[0042] The invention provides a method for detecting defects on the surface of strip steel based on deep learning. The method extracts local information on the surface of strip steel through a convolutional neural network, conducts comprehensive analysis in combination with a scale pyramid, and finally obtains the type of defect and its precise position at the same time. Such as Figure 5 , Figure 6 As shown, the type of defect is obtained by analyzing the principal components of the defect, and the precise location is obtained by visualization of the heat map.
[0043] Convolutiona...
PUM
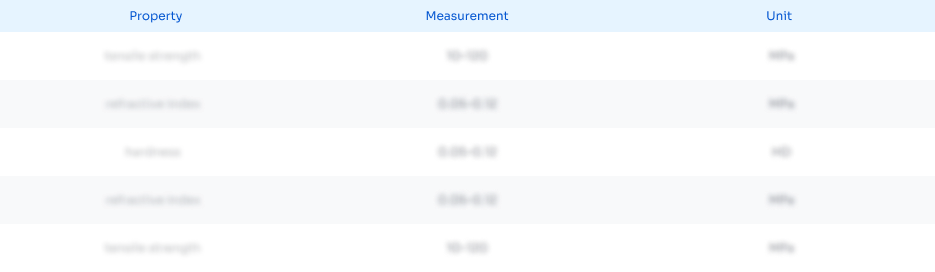
Abstract
Description
Claims
Application Information
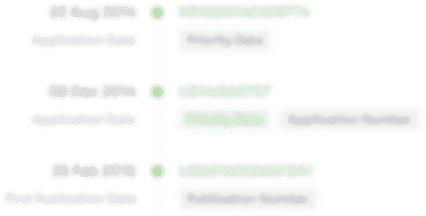
- R&D
- Intellectual Property
- Life Sciences
- Materials
- Tech Scout
- Unparalleled Data Quality
- Higher Quality Content
- 60% Fewer Hallucinations
Browse by: Latest US Patents, China's latest patents, Technical Efficacy Thesaurus, Application Domain, Technology Topic, Popular Technical Reports.
© 2025 PatSnap. All rights reserved.Legal|Privacy policy|Modern Slavery Act Transparency Statement|Sitemap|About US| Contact US: help@patsnap.com