Near-field signal source positioning method based on deep neural network regression model
A deep neural network and regression model technology, applied in biological neural network models, neural learning methods, positioning, etc., can solve the problems of lack of physical interpretation of classification models, low classification accuracy, and failure to meet accuracy requirements, etc., to achieve the minimum calculation cost, Estimated effect is good and the effect of reducing training cost
- Summary
- Abstract
- Description
- Claims
- Application Information
AI Technical Summary
Problems solved by technology
Method used
Image
Examples
Embodiment Construction
[0062] In order to make the purpose, technical effects and technical solutions of the embodiments of the present invention more clear, the technical solutions in the embodiments of the present invention are clearly and completely described below in conjunction with the accompanying drawings in the embodiments of the present invention; obviously, the described embodiments It is a part of the embodiment of the present invention. Based on the disclosed embodiments of the present invention, other embodiments obtained by persons of ordinary skill in the art without making creative efforts shall all fall within the protection scope of the present invention.
[0063] Data model and problem description:
[0064] Consider K near-field narrowband incoherent signals {s k (n)} is incident on a uniform linear array of M sensors with spacing d, and the sensors are assumed to be perfectly calibrated.
[0065] Set the center array element of the array as the phase reference point, then the ...
PUM
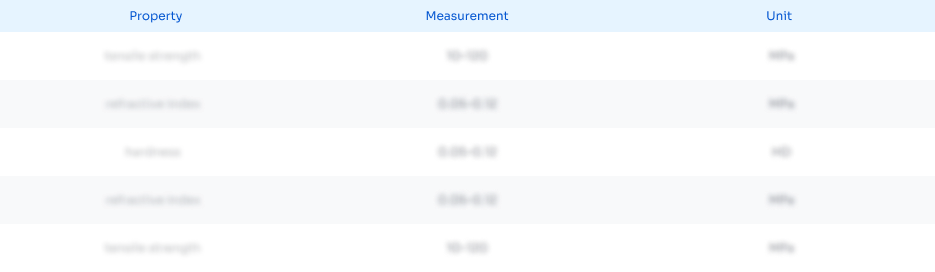
Abstract
Description
Claims
Application Information
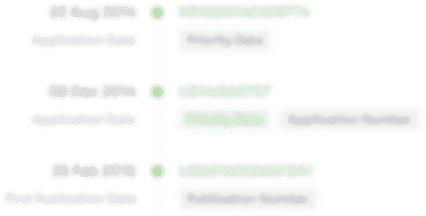
- R&D Engineer
- R&D Manager
- IP Professional
- Industry Leading Data Capabilities
- Powerful AI technology
- Patent DNA Extraction
Browse by: Latest US Patents, China's latest patents, Technical Efficacy Thesaurus, Application Domain, Technology Topic.
© 2024 PatSnap. All rights reserved.Legal|Privacy policy|Modern Slavery Act Transparency Statement|Sitemap