High-precision face recognition method based on deep transfer learning
A technology of face recognition and transfer learning, applied in character and pattern recognition, instruments, biological neural network models, etc., can solve problems such as slow convergence speed, harshness, difficulty in balancing recognition accuracy and model training speed, and achieve strong adaptability Effect
- Summary
- Abstract
- Description
- Claims
- Application Information
AI Technical Summary
Problems solved by technology
Method used
Image
Examples
Embodiment Construction
[0023] The present invention will be described in detail below in conjunction with the accompanying drawings and embodiments.
[0024] Such as figure 1 As shown, the present invention provides a high-precision face recognition method based on deep transfer learning technology, which consists of three parts, including an initial face recognition model unit, a target face recognition model unit, and a classifier unit.
[0025] The function of the initial face recognition model unit is to quickly improve the accuracy of face recognition, so that the classification loss quickly converges to a smaller value. The unit uses 160×160 RGB face color images as the input of the deep convolutional neural network, and selects a medium-scale face dataset as the training dataset (80,000 people, 5 million pictures). In order to be able to converge quickly, the SoftMax classification loss function is selected as the Loss Function of the unit. Using the gradient descent method to optimize the ...
PUM
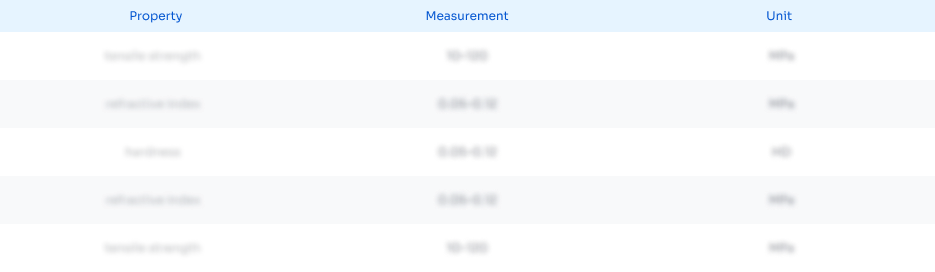
Abstract
Description
Claims
Application Information
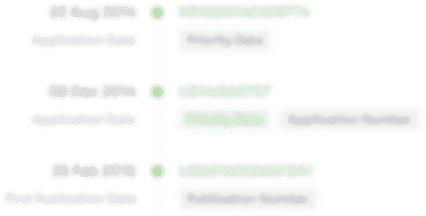
- R&D Engineer
- R&D Manager
- IP Professional
- Industry Leading Data Capabilities
- Powerful AI technology
- Patent DNA Extraction
Browse by: Latest US Patents, China's latest patents, Technical Efficacy Thesaurus, Application Domain, Technology Topic, Popular Technical Reports.
© 2024 PatSnap. All rights reserved.Legal|Privacy policy|Modern Slavery Act Transparency Statement|Sitemap|About US| Contact US: help@patsnap.com