Short-time parking demand prediction method based on GRU model
A demand forecasting and model technology, applied in neural learning methods, biological neural network models, traffic flow detection, etc., can solve the problem that the accuracy cannot meet the requirements of parking demand forecasting in parking lots, so as to avoid unnecessary traffic and improve service levels , the effect of high prediction accuracy
- Summary
- Abstract
- Description
- Claims
- Application Information
AI Technical Summary
Problems solved by technology
Method used
Image
Examples
Embodiment
[0067] Such as figure 1 As shown, a short-term parking demand prediction method based on the GRU model includes the following process: First, obtain the historical data of the parking lot facilities, process the historical data, and obtain the berth occupancy data at each time point; secondly, use deep learning Keras framework package, set the GRU neural network structure, use the GridSearch function in the Keras package to obtain the optimal parameters; finally, use the training set data to train the GRU model, save the model and predict the berth occupancy rate of the next step. In the embodiment, the GRU cell structure is as figure 2 shown. The input of the cell structure is the output value h of the cell at the previous moment t-1 And the observed berth occupancy value x at this moment t , z in the figure t with r t Indicate the update gate and reset gate respectively. The update gate is used to control the degree to which the state information at the previous moment...
PUM
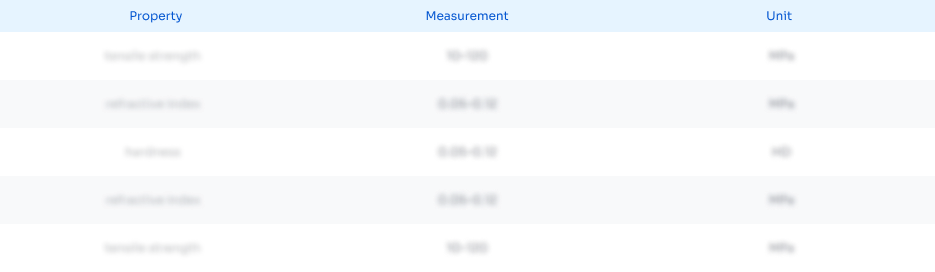
Abstract
Description
Claims
Application Information
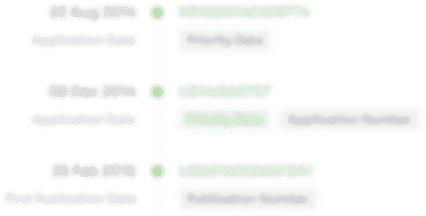
- R&D Engineer
- R&D Manager
- IP Professional
- Industry Leading Data Capabilities
- Powerful AI technology
- Patent DNA Extraction
Browse by: Latest US Patents, China's latest patents, Technical Efficacy Thesaurus, Application Domain, Technology Topic, Popular Technical Reports.
© 2024 PatSnap. All rights reserved.Legal|Privacy policy|Modern Slavery Act Transparency Statement|Sitemap|About US| Contact US: help@patsnap.com