Space-time attention mechanism method for traffic prediction
A traffic forecasting and attention technology, applied in forecasting, neural learning methods, data processing applications, etc., can solve the problems of time and external feature dependencies that cannot fully simulate spatial correlation.
- Summary
- Abstract
- Description
- Claims
- Application Information
AI Technical Summary
Problems solved by technology
Method used
Image
Examples
Embodiment Construction
[0074] The technical solutions of the present invention will be further described below in conjunction with specific embodiments and accompanying drawings.
[0075] A spatio-temporal attention mechanism method for traffic prediction, the steps are as follows:
[0076] The first step is to preprocess the traffic data
[0077] (1) Time granularity division: the entire time period (for example, one month) of all traffic data is divided into equal-length continuous time intervals.
[0078] (2) Spatial granularity division: define a group of road sections as A={1, 2, . . . , N}, where N represents the number of road sections.
[0079] (3) Standardize the data: Indicates the traffic volume of N road segments at time t. Given historical observations x=(x 1 ,x 2 ,...,x T )∈R N*T , traffic forecasting aims to predict Among them, T represents the sum of time, and h is the criterion for paying attention to different tasks;
[0080] In the second step, the preprocessed traffic da...
PUM
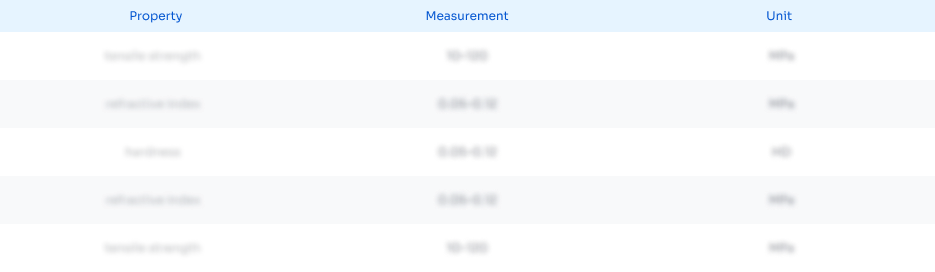
Abstract
Description
Claims
Application Information
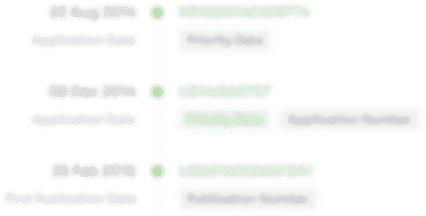
- R&D
- Intellectual Property
- Life Sciences
- Materials
- Tech Scout
- Unparalleled Data Quality
- Higher Quality Content
- 60% Fewer Hallucinations
Browse by: Latest US Patents, China's latest patents, Technical Efficacy Thesaurus, Application Domain, Technology Topic, Popular Technical Reports.
© 2025 PatSnap. All rights reserved.Legal|Privacy policy|Modern Slavery Act Transparency Statement|Sitemap|About US| Contact US: help@patsnap.com