Electromyographic signal gesture recognition method based on double-flow network
A technology of myoelectric signal and gesture recognition, which is applied in the field of human-computer interaction and artificial intelligence, can solve the problems of neglecting time correlation, etc., and achieve the effect of increasing recognition accuracy, improving recognition accuracy, and reasonable design
- Summary
- Abstract
- Description
- Claims
- Application Information
AI Technical Summary
Problems solved by technology
Method used
Image
Examples
Embodiment Construction
[0031] The technical solution of the present invention will be further described below in conjunction with the accompanying drawings.
[0032] A kind of myoelectric signal gesture recognition method based on double stream network, comprises the steps:
[0033] Step 1. Use figure 1 A set of non-invasive wearable EMG collection equipment is shown to collect 8 healthy volunteers as figure 2 The sEMG data of a total of five gestures are shown, and a total of 240 sEMG samples were obtained. Each sample contains 195 frames of EMG data. Each frame of EMG consists of 300 milliseconds of sEMG, and the acquisition device has 16 electrode channels, so the dimension of EMG is 300×16.
[0034] Step 2. According to image 3 As shown, a two-stream network model is established, which is mainly composed of a multi-layer CNN and a multi-layer LSTM. There are five layers in the CNN part of the model: the first two layers are convolutional layers, which contain 64 convolution kernels (5×5, ...
PUM
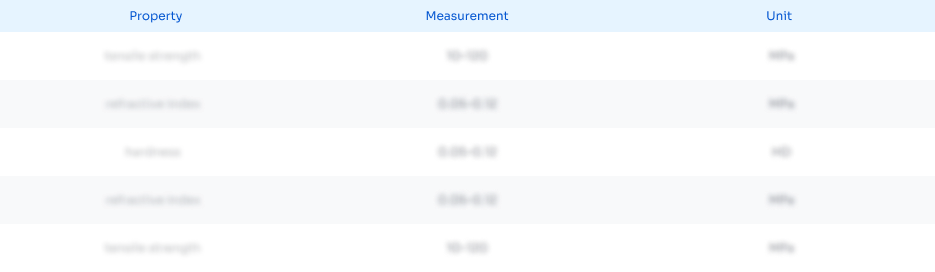
Abstract
Description
Claims
Application Information
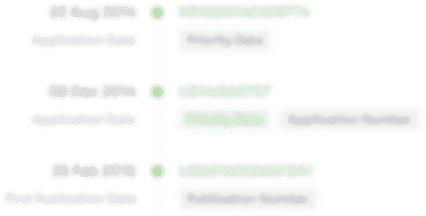
- R&D Engineer
- R&D Manager
- IP Professional
- Industry Leading Data Capabilities
- Powerful AI technology
- Patent DNA Extraction
Browse by: Latest US Patents, China's latest patents, Technical Efficacy Thesaurus, Application Domain, Technology Topic, Popular Technical Reports.
© 2024 PatSnap. All rights reserved.Legal|Privacy policy|Modern Slavery Act Transparency Statement|Sitemap|About US| Contact US: help@patsnap.com