Multi-scale target tracking method based on learning rate adjustment and multi-layer convolution feature fusion
A technology of target tracking and learning rate, which is applied in the field of multi-scale target tracking based on learning rate adjustment and fusion of multi-layer convolution features, can solve problems such as blurring, lack of semantic information, and affecting classifier performance, so as to improve accuracy and robustness strong effect
- Summary
- Abstract
- Description
- Claims
- Application Information
AI Technical Summary
Problems solved by technology
Method used
Image
Examples
Embodiment Construction
[0032] In order to have a clearer understanding of the technical features, purposes and effects of the present invention, the specific implementation manners of the present invention will now be described with reference to the accompanying drawings.
[0033] figure 1 It is the method flowchart of the present invention:
[0034] Step 1: Obtain the initial position information and scale information of the target.
[0035] Step 2: Extract the convolutional features of the target based on the initial information obtained in the first step.
[0036] By establishing the minimum cost function to train a position filter for each layer of neural network, the optimal correlation filter is obtained:
[0037]
[0038] In formula (1), f l Is a d-dimensional feature vector, d represents the dimension of the selected feature, l∈{1,2,...d}. The filter corresponding to each channel is h l , * means convolution operation, superscript l means a certain dimension of the feature, g means t...
PUM
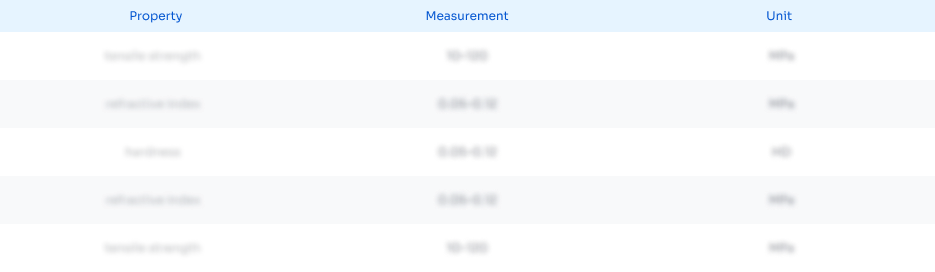
Abstract
Description
Claims
Application Information
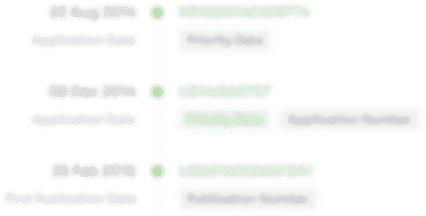
- R&D Engineer
- R&D Manager
- IP Professional
- Industry Leading Data Capabilities
- Powerful AI technology
- Patent DNA Extraction
Browse by: Latest US Patents, China's latest patents, Technical Efficacy Thesaurus, Application Domain, Technology Topic, Popular Technical Reports.
© 2024 PatSnap. All rights reserved.Legal|Privacy policy|Modern Slavery Act Transparency Statement|Sitemap|About US| Contact US: help@patsnap.com