Unsupervised cross-modal hash retrieval method and system based on virtual label regression
A virtual label and cross-modal technology, applied in the field of cross-modal retrieval, can solve problems such as large-scale errors, limited semantic information, and limited expression ability of hash codes, so as to reduce quantization errors, improve performance, and ensure semantic consistency Effect
- Summary
- Abstract
- Description
- Claims
- Application Information
AI Technical Summary
Problems solved by technology
Method used
Image
Examples
Embodiment 1
[0041] Such as Figure 1-2 As shown, Embodiment 1 of the present disclosure provides an unsupervised cross-modal hash retrieval method based on virtual tag regression, including:
[0042] S1: Obtain cross-modal retrieval datasets and divide them into training set, test set and database set, where each sample includes paired image and text data of two modalities.
[0043] The training set has n samples, and each sample includes paired image and text modal data. The image feature matrix of the sample is expressed as d 1 Represents the dimension of image features, and the text feature matrix of samples is expressed as d 2 represents the dimensionality of text features, and the goal is to learn a shared hash code B ∈ [-1,1] n×r , r represents the length of the hash code.
[0044] S2: Build a deep hash model and initialize network parameters.
[0045] A deep hash network consists of two parts. For the image modality, the VGG-16 model is adopted as the basic deep hashing n...
Embodiment 2
[0103] Embodiment 2 of the present disclosure provides an unsupervised deep cross-modal hash retrieval system based on virtual label regression, including:
[0104] The image preprocessing module is configured to: obtain a cross-modal retrieval data set, and divide them into a training set, a test set and a database set, wherein each sample includes paired image and text data;
[0105] The network model building module is configured to: build a deep hash model and initialize network parameters;
[0106] The depth feature matrix and deep network output acquisition module are configured to: respectively input the original data of the two modalities into the constructed deep hash network to obtain a depth feature matrix, and the depth feature matrix continues to be transmitted along the network, Get the output value of the deep hash network;
[0107] The objective function building module is configured to: according to the deep feature matrix of the training set, the virtual lab...
Embodiment 3
[0111] Embodiment 3 of the present disclosure provides a computer-readable storage medium on which a computer program is stored, which is characterized in that, when the program is executed by a processor, the unsupervised depth based on virtual label regression described in Embodiment 1 of the present disclosure is implemented. Cross-modal hash retrieval method.
PUM
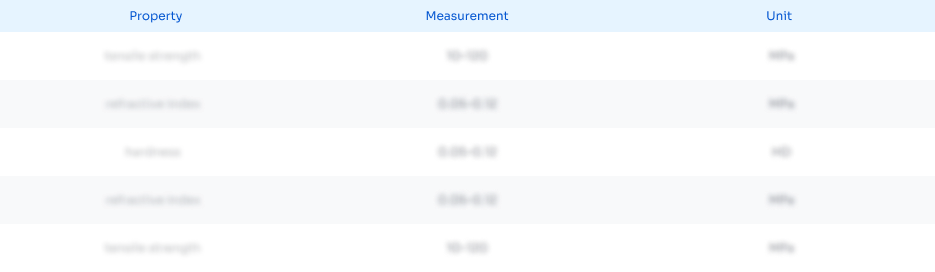
Abstract
Description
Claims
Application Information
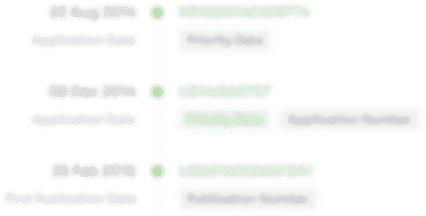
- R&D Engineer
- R&D Manager
- IP Professional
- Industry Leading Data Capabilities
- Powerful AI technology
- Patent DNA Extraction
Browse by: Latest US Patents, China's latest patents, Technical Efficacy Thesaurus, Application Domain, Technology Topic, Popular Technical Reports.
© 2024 PatSnap. All rights reserved.Legal|Privacy policy|Modern Slavery Act Transparency Statement|Sitemap|About US| Contact US: help@patsnap.com