Fast probabilistic power flow calculation method of improved extreme learning machine considering power flow characteristics
A technology of probabilistic power flow calculation and extreme learning machine, which is applied in the field of electric power system and its automation, can solve the problems of rising hidden layer parameter error and limited feature extraction ability of shallow structure, so as to improve fitting ability, reduce fitting difficulty, The effect of improving the fitting effect
- Summary
- Abstract
- Description
- Claims
- Application Information
AI Technical Summary
Problems solved by technology
Method used
Image
Examples
Embodiment 1
[0041] see Figure 1 to Figure 3 , an improved extreme learning machine fast probabilistic power flow calculation method considering power flow characteristics, mainly includes the following steps:
[0042] 1) Obtain the basic data of the power network.
[0043] Furthermore, the basic data of the electric power network mainly includes active power, reactive power, voltage amplitude, voltage phase angle, branch active power and reactive power of electric power network nodes.
[0044] 2) Based on the basic data of the power network, establish a mapping relationship f:P i , Q i → U i , θ i . Among them, P i , Q i Denote the active power and reactive power injected by node i, respectively. u i , θ i Respectively represent the voltage amplitude and voltage phase angle of node i.
[0045] 3) Decompose the mapping relationship and establish an extreme learning machine neural network.
[0046] Further, the main steps of establishing the extreme learning machine neural netw...
Embodiment 2
[0070] The improved extreme learning machine fast probabilistic power flow calculation method considering the characteristics of the power flow mainly includes the following steps:
[0071] 1) Obtain the basic data of the power network.
[0072] 2) Based on the basic data of the power network, establish a mapping relationship f:P i , Q i → U i , θ i . Among them, P i , Q i Denote the active power and reactive power injected by node i, respectively. u i , θ i represent nodes respectively i voltage amplitude and voltage phase angle.
[0073] 3) Decompose the mapping relationship and establish an extreme learning machine neural network.
[0074] 4) Optimize the hidden layer parameters of the extreme learning machine neural network, thereby establishing an improved extreme learning machine neural network.
[0075] 5) Input the basic data of the power network into the neural network of the improved extreme learning machine, and calculate the probability flow of the powe...
Embodiment 3
[0077] The main steps of the improved extreme learning machine fast probability power flow calculation method considering the characteristics of the power flow are shown in Example 2, wherein the main steps of establishing the extreme learning machine neural network are as follows:
[0078] 1) The mapping relationship f:P i , Q i → U i , θ i Decomposed into mapping relationship f 1 :P i , Q i →P ij , Q ij and the mapping relationship f 2 :P ij , Q ij → U i , θ i .
[0079] 2) Based on the mapping relationship f 1 :P i , Q i →P ij , Q ij , to establish the first-stage extreme learning machine neural network. Among them, the input layer is the active power P injected by node i i and the reactive power Q injected by node i i . The hidden layers include unsupervised layers with ELM sparse autoencoder, supervised layers with ELM original algorithm and supervised layers with error correction module. The output layer is the branch active power P of the line ij ...
PUM
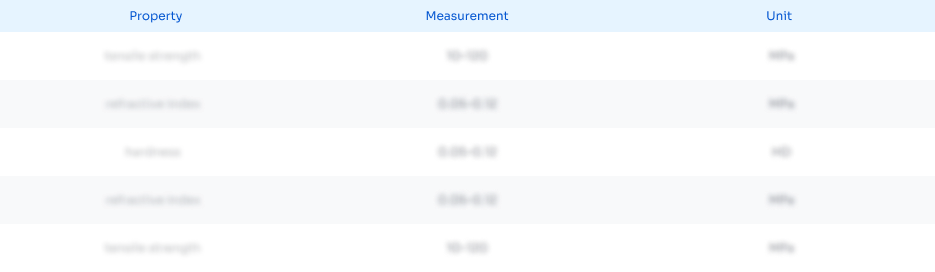
Abstract
Description
Claims
Application Information
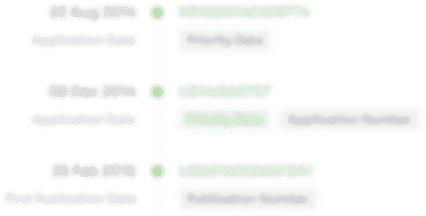
- Generate Ideas
- Intellectual Property
- Life Sciences
- Materials
- Tech Scout
- Unparalleled Data Quality
- Higher Quality Content
- 60% Fewer Hallucinations
Browse by: Latest US Patents, China's latest patents, Technical Efficacy Thesaurus, Application Domain, Technology Topic, Popular Technical Reports.
© 2025 PatSnap. All rights reserved.Legal|Privacy policy|Modern Slavery Act Transparency Statement|Sitemap|About US| Contact US: help@patsnap.com