Non-invasive load decomposition based on improved differential evolution algorithm
An improved differential evolution and non-invasive technology, applied in spectrum analysis/Fourier analysis, complex mathematical operations, measurement devices, etc., can solve problems such as inability to realize load equipment identification, low load feature identification, and sparse features
- Summary
- Abstract
- Description
- Claims
- Application Information
AI Technical Summary
Problems solved by technology
Method used
Image
Examples
Embodiment Construction
[0020] Step 1: Extract the steady-state current harmonic characteristics of typical household appliances and establish a characteristic sample set.
[0021] The second step: sample the power and current at the user's incoming line, and use the event detection algorithm to obtain the time section of the steady state operation of the load equipment.
[0022] The third step: Sample the currents in the same time segment, and use FFT to extract the harmonic characteristics of the steady-state current.
[0023] Step 4: Initialize the relevant parameters and set the first generation population {X 1,1 ,X 2,1 ,...,X n,1 }, size n, each individual X i Are all m-dimensional 0-1 vectors {x 1 ,x 2 ,...,X m }, where x m Is the operating state of the m-th load equipment.
[0024] Step 5: Perform mutation, crossover and selection operations according to DE (Differential Evolution Algorithm), and calculate fitness according to Equation 3.
[0025] Step 6: Determine whether the set number of iterations i...
PUM
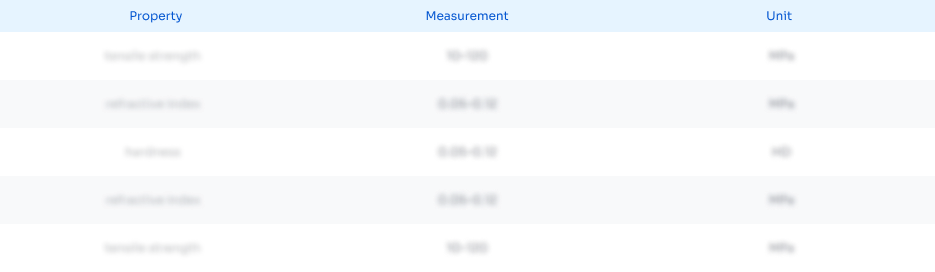
Abstract
Description
Claims
Application Information
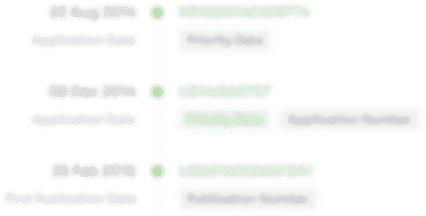
- R&D
- Intellectual Property
- Life Sciences
- Materials
- Tech Scout
- Unparalleled Data Quality
- Higher Quality Content
- 60% Fewer Hallucinations
Browse by: Latest US Patents, China's latest patents, Technical Efficacy Thesaurus, Application Domain, Technology Topic, Popular Technical Reports.
© 2025 PatSnap. All rights reserved.Legal|Privacy policy|Modern Slavery Act Transparency Statement|Sitemap|About US| Contact US: help@patsnap.com