Image recognition method based on nonlinear enhanced subspace clustering
An image recognition and subspace technology, applied in character and pattern recognition, instruments, computing, etc., can solve problems such as affecting the accuracy of image recognition, lack of local information, etc., to improve the effect of image recognition and improve the effect of image recognition.
- Summary
- Abstract
- Description
- Claims
- Application Information
AI Technical Summary
Problems solved by technology
Method used
Image
Examples
Embodiment 1
[0043] An image recognition method based on nonlinear augmented subspace clustering, such as figure 1 shown, including the following steps:
[0044] S1. Obtain an image data set; crop the images in the image data set to a uniform size; if the image data set contains a color image, perform dimensionality reduction processing on the color image;
[0045] The obtained image data set is denoted as X∈R (D*n) , D represents the dimension, and n is the total number of data points in the image data set;
[0046] S2. Solve the local linear expression matrix of the image data set by using a local linear embedding algorithm to extract the nonlinear manifold structure of the image data set:
[0047] Based on the KNN algorithm, according to the Euclidean distance as a measure, calculate the distance from the data point x in the image data set X i The nearest k nearest neighbors, if the j-th data point is the nearest neighbor of the i-th data point, then the i-th row and j-column of the ...
Embodiment 2
[0071] In this embodiment 2, a simulation experiment is carried out based on the image recognition method based on nonlinear enhanced subspace clustering provided in embodiment 1, which is specifically applied to face image recognition in this experiment. The experiment uses 5 data sets, including 3 face data sets: ORL, Yale Face, CMU-PIE data set; 2 object recognition data sets: COIL20, CIFAR-10 data set. The ORL contains 40 different subjects, and each object has 10 images taken in different situations, with different facial expressions, facial details and lighting conditions. The Yale Face dataset contains 165 images of 15 people. Each subject has 11 different images with different facial expressions and lighting conditions. CMU-PIE is a popular face dataset widely used in a variety of learning tasks. It includes 68 subjects, totaling 41,368 face images. The method in Example 1 is applied to object image clustering on COIL20 and CIFAR-10 respectively. The CIFAR-10 datas...
PUM
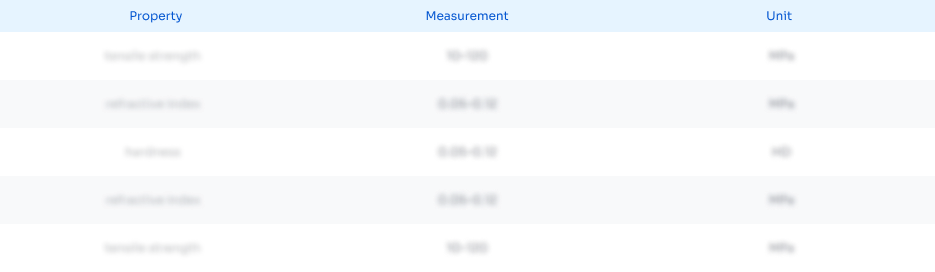
Abstract
Description
Claims
Application Information
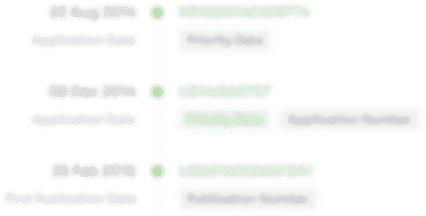
- R&D Engineer
- R&D Manager
- IP Professional
- Industry Leading Data Capabilities
- Powerful AI technology
- Patent DNA Extraction
Browse by: Latest US Patents, China's latest patents, Technical Efficacy Thesaurus, Application Domain, Technology Topic, Popular Technical Reports.
© 2024 PatSnap. All rights reserved.Legal|Privacy policy|Modern Slavery Act Transparency Statement|Sitemap|About US| Contact US: help@patsnap.com