A block patch reconstruction method for point cloud data based on deep learning
A technology of point cloud data and deep learning, which is applied in the field of block face reconstruction of point cloud data, can solve problems such as result errors, achieve the effects of avoiding geometric operations, powerful fitting capabilities, and improving reliability
- Summary
- Abstract
- Description
- Claims
- Application Information
AI Technical Summary
Problems solved by technology
Method used
Image
Examples
Embodiment Construction
[0025] The present invention will be described in detail below according to the accompanying drawings.
[0026] refer to figure 1 , the deep convolutional neural network used in the present invention needs to use the voxel information converted from the point cloud as input, and output the corresponding SDF. Therefore, during training, this method prepares the matching voxel information and SDF as the data set for network training. This method uses the original patch data in the A Benchmark for 3D Mesh Segmentation dataset open sourced by Princeton University to calculate the corresponding point cloud data containing normal information, and generate the corresponding SDF. Randomly select a point in the generated point cloud as the center of the circle, search for adjacent points in the fixed-size radius and generate voxels as the input data of the deep convolutional neural network, and then select SDF in the corresponding area as the true value for training .
[0027] figur...
PUM
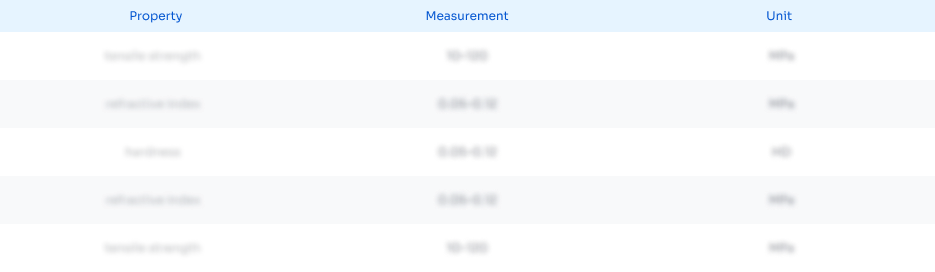
Abstract
Description
Claims
Application Information
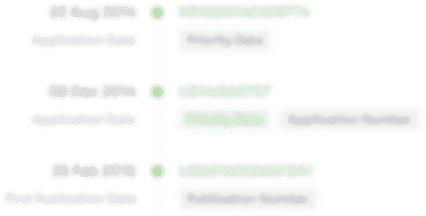
- R&D Engineer
- R&D Manager
- IP Professional
- Industry Leading Data Capabilities
- Powerful AI technology
- Patent DNA Extraction
Browse by: Latest US Patents, China's latest patents, Technical Efficacy Thesaurus, Application Domain, Technology Topic.
© 2024 PatSnap. All rights reserved.Legal|Privacy policy|Modern Slavery Act Transparency Statement|Sitemap