Semi-supervised generative adversarial network image classification method based on local manifold regularization
A network image and classification method technology, applied in the field of image semi-supervised classification, can solve the problems of model collapse and failure to use regularization methods, etc., and achieve the effect of strong fitting ability and strong construction ability
- Summary
- Abstract
- Description
- Claims
- Application Information
AI Technical Summary
Problems solved by technology
Method used
Image
Examples
Embodiment Construction
[0039] The technical solutions in the embodiments of the present invention will be described clearly and in detail below with reference to the drawings in the embodiments of the present invention. The described embodiments are only some of the embodiments of the invention.
[0040] The technical scheme that the present invention solves the problems of the technologies described above is:
[0041] Such as figure 1 and figure 2 As shown, the specific steps of the semi-supervised generation confrontation network image classification method combined with local manifold regularization in the present invention are:
[0042] Step 1: Create an image dataset, label a part of the data, record the total category of the image as N, and define a labeled dataset where x i is the image, y i for the corresponding label. Define an unlabeled dataset The image data set is divided into training set and test set, the training set is used to train the semi-supervised classification model,...
PUM
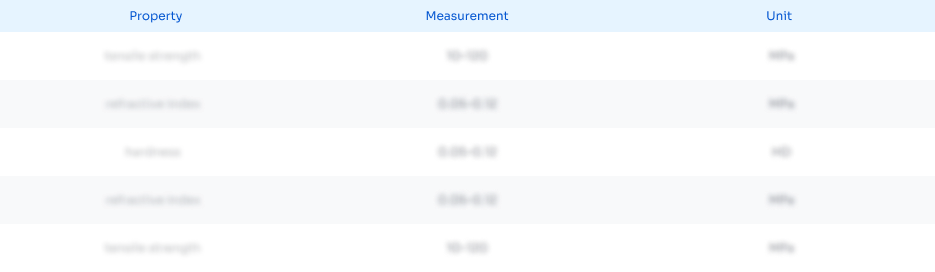
Abstract
Description
Claims
Application Information
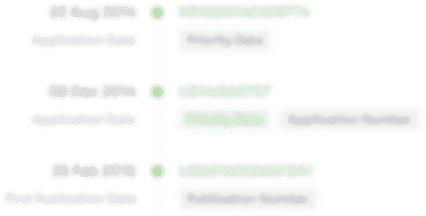
- Generate Ideas
- Intellectual Property
- Life Sciences
- Materials
- Tech Scout
- Unparalleled Data Quality
- Higher Quality Content
- 60% Fewer Hallucinations
Browse by: Latest US Patents, China's latest patents, Technical Efficacy Thesaurus, Application Domain, Technology Topic, Popular Technical Reports.
© 2025 PatSnap. All rights reserved.Legal|Privacy policy|Modern Slavery Act Transparency Statement|Sitemap|About US| Contact US: help@patsnap.com