Medical information cross-modal hash coding learning method based on generative adversarial network
A hash coding, medical information technology, applied in character and pattern recognition, image analysis, image enhancement and other directions, can solve the problem of not making full use of semantic information, unable to achieve semantic association and so on
- Summary
- Abstract
- Description
- Claims
- Application Information
AI Technical Summary
Problems solved by technology
Method used
Image
Examples
Embodiment 1
[0043] Embodiment 1: as Figure 1-4 As shown, a method for learning cross-modal hash coding of medical information based on generative confrontation network, the specific steps of the method are as follows:
[0044] Step1. Perform feature extraction on the chest CT image-text data; first perform chest CT image preprocessing, cut out the ROI image block, and then extract images from the ROI image block and chest CT image-text data through the CMSFF model and the bag-of-words model features and textual features;
[0045] Further, the specific steps of the Step1 are as follows:
[0046] Step1.1, firstly perform data preprocessing; for the image data set, in order to avoid the loss of pixels caused by direct compression from the size of 512*512 to 224*224, the method of cutting the original CT image is adopted; according to the lung nodules on the slice The diameters are different, and the Region Of Interest, ROI image block with the size R={16*16, 32*32, 64*64, 128*128} is cut ...
Embodiment 2
[0080] Embodiment 2: as Figure 1-5 As shown, a kind of medical information cross-modal hash coding learning method based on generative confrontation network, this embodiment is the same as embodiment 1, the difference is:
[0081] Among them, Step6, the hash codes of different modalities learned through Step5 are stored in the hash code database, and at the same time, a trained model is obtained for the cross-modal retrieval system. Input the text data of any group of pulmonary nodules, obtain the corresponding hash code through cross-modal retrieval, and then retrieve the optimal result in the hash code database. The retrieval process is as follows: figure 2 shown.
[0082] The concrete steps of described Step6 are:
[0083] For the text mode, let its input be y, and learn its hash code through the GANHL model, such as the formula C y = h (y) (f (y) (y; θ t , θ D )) shown.
[0084] The query data of the given text is realized through the GANHL retrieval model, and t...
PUM
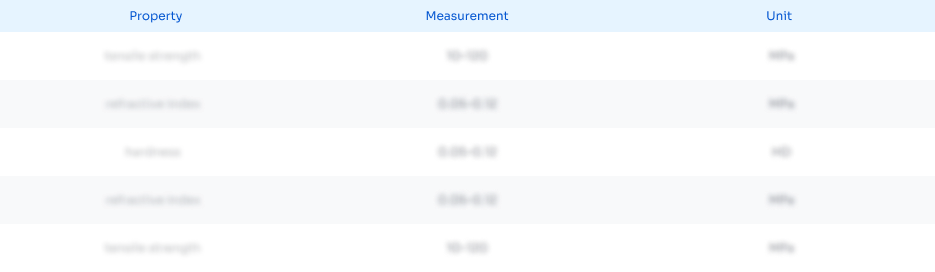
Abstract
Description
Claims
Application Information
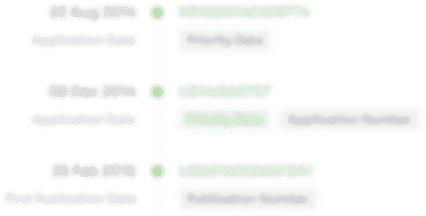
- R&D Engineer
- R&D Manager
- IP Professional
- Industry Leading Data Capabilities
- Powerful AI technology
- Patent DNA Extraction
Browse by: Latest US Patents, China's latest patents, Technical Efficacy Thesaurus, Application Domain, Technology Topic, Popular Technical Reports.
© 2024 PatSnap. All rights reserved.Legal|Privacy policy|Modern Slavery Act Transparency Statement|Sitemap|About US| Contact US: help@patsnap.com