Multi-modal brain image depression identification method and system based on graph node embedding
A recognition method and brain imaging technology, applied in the field of brain neuroscience, can solve the problems of not achieving good results and low classification accuracy.
- Summary
- Abstract
- Description
- Claims
- Application Information
AI Technical Summary
Problems solved by technology
Method used
Image
Examples
Embodiment Construction
[0031] Embodiments of the present invention will be described in further detail below in conjunction with the accompanying drawings.
[0032] A multimodal brain image depression recognition method based on graph node embedding, comprising the following steps:
[0033] 1) Obtain resting-state fMRI and DTI image data of depressed patients and normal controls;
[0034] 2) Preprocessing the acquired fMRI and DTI image data;
[0035] 3) According to the preprocessed fMRI and DTI image data, the brain functional network and structural network are respectively constructed to obtain the brain network adjacency matrix;
[0036] 4) Using graph node embedding to represent the adjacency matrix as an image, input it into a convolutional neural network for classification, and establish a classification model for identifying depressed patients and normal subjects.
[0037] Such asfigure 1 Shown is the flow chart of the preprocessing and network construction of the original fMRI and DTI dat...
PUM
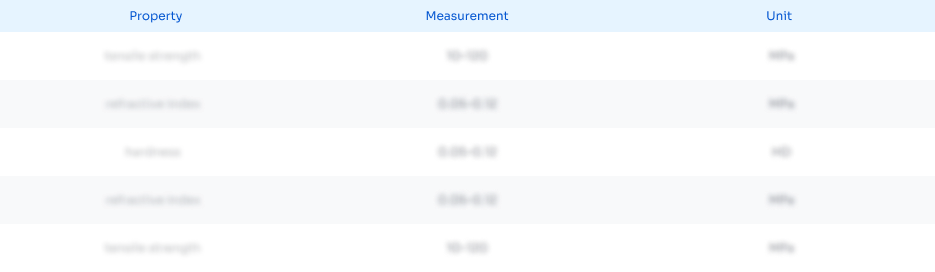
Abstract
Description
Claims
Application Information
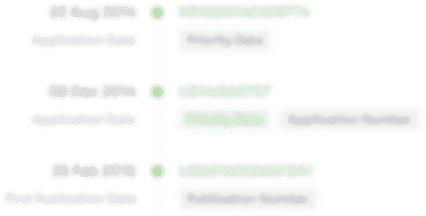
- R&D Engineer
- R&D Manager
- IP Professional
- Industry Leading Data Capabilities
- Powerful AI technology
- Patent DNA Extraction
Browse by: Latest US Patents, China's latest patents, Technical Efficacy Thesaurus, Application Domain, Technology Topic, Popular Technical Reports.
© 2024 PatSnap. All rights reserved.Legal|Privacy policy|Modern Slavery Act Transparency Statement|Sitemap|About US| Contact US: help@patsnap.com