Image denoising method based on residual learning and convolutional neural network
A convolutional neural network and image technology, applied in the field of image processing, can solve the problems of increased training difficulty, reduced training effect, and the inability of convolutional neural networks to maintain image structure information, so as to improve the learning effect, avoid loss and Loss, the effect of improving the retention effect
- Summary
- Abstract
- Description
- Claims
- Application Information
AI Technical Summary
Problems solved by technology
Method used
Image
Examples
Embodiment Construction
[0033] The present invention will be further described in detail below in conjunction with specific implementation methods and accompanying drawings.
[0034] Such as figure 1 As shown, the image denoising method based on residual learning and convolutional neural network of the present invention comprises the following steps:
[0035] (1) Use the convolutional neural network method to denoise the input noisy image, and construct a convolutional neural network model, including multiple convolutional layers; the number of convolution kernels in each layer of the convolutional neural network model is the same And the size is consistent, all use 3×3 convolution kernels for convolution, and the number of convolution kernels in each layer is 64; the convolution layer in the convolutional neural network model uses the ReLU activation function;
[0036] (2) Use the method of residual learning to denoise the input noisy image, superimpose a network mapping layer on a neural network, ...
PUM
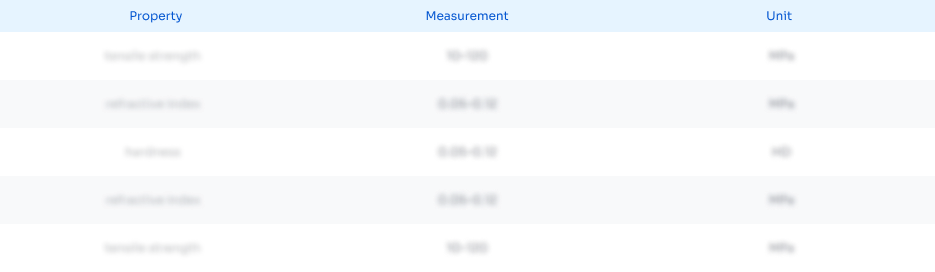
Abstract
Description
Claims
Application Information
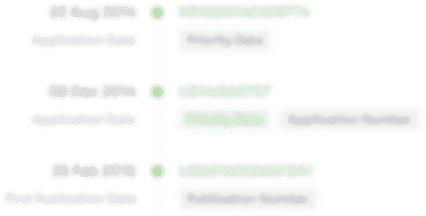
- R&D Engineer
- R&D Manager
- IP Professional
- Industry Leading Data Capabilities
- Powerful AI technology
- Patent DNA Extraction
Browse by: Latest US Patents, China's latest patents, Technical Efficacy Thesaurus, Application Domain, Technology Topic, Popular Technical Reports.
© 2024 PatSnap. All rights reserved.Legal|Privacy policy|Modern Slavery Act Transparency Statement|Sitemap|About US| Contact US: help@patsnap.com